Correlation of digital health use and chronic pain coping strategies
Introduction
“Digital health”, defined as the convergence of digital technologies and healthcare, is widely promoted for patient engagement (1,2). Some digital health tools—such as websites, mobile applications, and personal health monitoring devices—have demonstrated efficacy at improving medication adherence, management of chronic conditions, and patient safety (3-7). Studies suggest that digital health is most often used by patients seeking additional information or support (8).
Chronic pain is common. Patients with chronic pain articulate frustrations about lack of engagement in their healthcare; for instance, qualitative research suggests that comprehension of patients’ individual circumstances and access to evidence about treatment options are challenges for many patients with chronic pain (9). Effective application of chronic pain coping techniques may result in lower pain and improved health outcomes (10). Digital health may be salient for patients with chronic pain-related diagnoses by overcoming barriers to care such as: access to non-pharmaceutical therapies; pain-related mobility issues; peer networking and support; awareness of alternative diagnoses and therapeutic options (11-14). It may thereby provide a possible approach for increasing patient engagement and coping skills.
It is unknown, however, whether digital health use improves coping techniques, particularly among chronic pain patients. Existing chronic pain-focused mobile applications are overly simplistic, rarely based in theory, and rarely supported by evidence (15). Little research has been done to catalogue the use of digital health in the chronic pain patient population, or to describe whether its use is associated with pain-related coping skills.
This brief report aims to describe patterns of digital health usage among chronic pain patients engaged in a popular online patient discussion forum, and to examine whether digital health use correlates with health care utilization and pain-related coping skills.
Methods
Study setting
This cross-sectional survey was administered to patients with a self-identified chronic pain diagnosis participating in ‘Patients Like Me’® (PLM), an organization that directly collects data from patients experiencing chronic health conditions, with emphasis on patient-centered outcomes and experiences interacting with the health care system. PLM has a specified goal of sharing these data with researchers to increase healthcare research by decreasing barriers to accessing patient experiences (16). Independent institutional review board approval was obtained for the study.
Participant recruitment
PLM sent out invitations to complete the survey to members who met the following eligibility criteria: age 21–64 years, residing in the US, had at least one log-in to the PLM site in the past 30 days, owned or had access to a cell phone, reported pain as a condition on their profile or had experienced chronic pain in the past 12 months. All eligible users were invited via email, by PLM, to participate in the survey. The initial survey invite was followed by an email reminder if the user had not responded within two weeks. Sampling was conducted with the goal of recruiting a survey sample of at least 15% non-White race or Hispanic or Latino ethnicity, no more than 60% female, and no less than 40% with some college or less education. The email invites for participation in the study were sent in three waves to patient panels that would help meet the predetermined sampling goals, based on PLM's internal knowledge of their membership. Each wave included 500 invitations.
Survey development
The survey consisted of validated and piloted measures relevant to chronic pain. Specific measures analyzed for this manuscript included:
- Demographic information: questions about gender, race, ethnicity, education, employment and insurance status were asked. These questions and responses were adopted from similar demographic questions previously administered across multiple prior studies.
- Frequency of health service use was measured using items from the National Health Interview Survey (17), asking about number of visits in the past 12 months to a primary care clinic or doctor’s office or to a hospital emergency department. Response options ranged from not used in the past 12 months to daily or almost daily use.
- Pain coping was measured using the Chronic Pain Coping Inventory (CPCI) 26-item wellness subscales (which contains measures of four specific wellness-related coping strategies) (18). This is a licensed assessment scale, with demonstrated reliability and validity (19). Wellness subscale items asked respondents on how many days in the past week they had used a list of 26 strategies to cope with their pain (response options ranged from 0 to 7 days). The items were summed and averaged to generate an overall score, as well as subscale scores for each of the wellness-related pain coping strategies: self-talk, relaxation, task persistence, exercise/stretching, and seeking social support. Higher scores correlate with better coping skills.
- Use of digital technology: respondents indicated their ownership or access to technology (such as cell-phones and computers), as well as their self-reported use of online medical resources, social media and mobile phone applications in managing their pain and health. Options for use were: before a visit to the healthcare provider, during the visit, after the visit, or no use of the technology in this way. These questions were adapted from a previously piloted survey used with emergency department patients (20). Digital health use was described in terms of types of digital health use (website, social media, mobile applications) as well as temporal use of digital health. Digital health use “of any kind” was defined as a positive response to the use of websites, social media, or mobile applications at any time point to inform health care.
Analysis
De-identified survey responses were transferred from the PLM database to a secure study database prior to analysis. Descriptive statistics were calculated for all variables, with continuous data reported as means with standard deviations, and proportions reported with 95% confidence intervals. To examine correlates of low (0 to 1 form of digital health used at any point in care) vs. high (2+ forms of digital health used at any point in care) digital health use, univariable logistic regression was conducted to examine its association with patient characteristics; multivariable logistic regression was then run, with level of digital health use as the dependent variable, adjusting for significant variables on the univariable regression. Finally, linear regression was conducted to explore differences in pain coping styles (for each of the CPCI wellness subscales) by digital health use, adjusting for gender and age, and including an interaction term between digital health use and age older than 50 years.
Results
A total 565 responses were obtained (122 in October 2014, 240 in January 2015, 203 in March 2015) through three waves of invitations from PLM (response rate 37.6%). Characteristics of the participants are given in Table 1. The mean age of the study population was 51.3 (SD =10.8). Most respondents were female (87.2%), non-Hispanic (95.1%), and white (88.1%). A minority of respondents reported having completed college (37.3%), being employed (33.8%), or having private insurance (45.7%). Further descriptive characteristics are in Table 1. Data on non-respondents was unavailable.
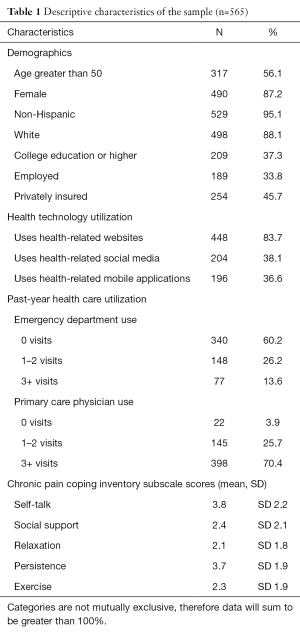
Full table
Most participants (89.5%) reported some digital health use (see Table 2). Websites were the most common form of health-related technology used (84.1%), and “apps” the least (39.4%). Although few respondents (11.8%) reported using digital health during a healthcare visit, those who did most often reported using apps (6.5%). Almost half (48.5%) reported being high utilizers of digital health (using more than one form, per our a priori definition).
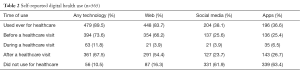
Full table
In the unadjusted analysis, females and participants aged <50 years old were more likely to be high utilizers of digital health (see Table 3). No significant association was found between high digital health use and other demographic factors, nor between digital health use and healthcare utilization.
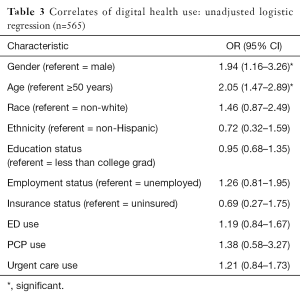
Full table
On multivariable linear regression, high digital health use predicted higher scores for the support (intercept 1.33; β=0.53; 95% CI, 0.05–1.01; P=0.03), relaxation (intercept 1.49; β=0.53; 95% CI, 0.12–0.94; P=0.01), and exercise (intercept 2.08; β=0.54; 95% CI, 0.10–0.98; P=0.016) subscales; it did not predict higher scores on the self-talk subscale (intercept 2.81; β=0.50; 95% CI, −0.02–1.01; P=0.06) or the persistence subscale (intercept 3.80; β=0.06; 95% CI, −0.39–0.51; P=0.26). Female gender significantly predicted both higher self-talk subscale scores (β=0.96; 95% CI, 0.39–1.53; P=0.001) and relaxation scores (β=0.46; 95% CI, 0.01–0.92; P=0.05). The interaction term between age <50 years and high digital health use indicated lower coping self-talk subscale scores β=−0.80; 95% CI, −1.57– −0.02; P=0.05) among this subgroup. Age group alone did not predict any of the CPCI subscale scores. A sensitivity analysis including a gender x digital health interaction score did not change the results appreciably, worsened model fit, and was therefore dropped.
Discussion
This cross-sectional study of a predominantly low-income, female population experiencing chronic pain provides preliminary data supporting digital health to enhance chronic pain coping mechanisms.
Almost 90% of respondents, across demographic groups, reported use of some form of digital health. In accordance with national data, the most commonly used form was websites, but apps and social media were used by over 1/3 of respondents. This survey did not explore whether this population uses apps and social media for non-health-related purpose. Others’ work suggests that the more comfortable a patient is with a form of technology, the more likely she or he is with using that technology type to facilitate health (21,22).
All three forms of digital health were likely to be used before and after a healthcare appointment, but rarely during an appointment. These differences in usage patterns may reflect prevalent physician discomfort with, or lack of knowledge about, digital health (23-25). It is possible that opportunities exist for physicians to use digital health to enhance patient engagement during a healthcare visit. Future work should explore the utility of digital health use during a visit for patients with chronic pain diagnoses, and whether enhanced usage during an appointment would be feasible, acceptable, and effective.
In accordance with others’ and our own work, younger age, but not education level or socioeconomic status, correlated with use of digital health by this population. Others’ work suggests that elderly patients may have decreased comfort with technology; this disparity in usage may, however, be disappearing (26-28). Indeed, our findings support the idea that a socioeconomic “digital gap” is decreasing (if existent at all) and that digital health interventions are acceptable and used among all strata of society.
Supporting the initial study hypothesis, we observed that using more forms of digital correlated with higher levels of many well-established chronic pain coping techniques, including support, relaxation, and exercise skills. These three coping techniques were employed the least frequently by respondents; it is therefore possible that digital health has a greater influence on skills which do not already exist. Alternatively, these coping skills may be most amenable to the influence of digital health. Indeed, others’ work suggests that multiple forms of digital health—ranging from Twitter to online curricula—can be effective in increasing exercise, encouraging mindfulness, and increasing distress tolerance. Some theorize that these findings are largely due to the social support environment (12).
Provocatively, patients who were both younger (<50 years old) and using multiple forms of digital health had lower self-talk coping skills. Neither digital health use nor any demographic factors predicted persistence skillsets. Patients with multiple chronic conditions may perceive digital health resources as an extra burden, and possibly even as a negative influence on mood and health status (29). It is also possible that the development of self-talk and persistence skills—often addressed through techniques such as cognitive behavioral therapy—may require more time, practice, and formal instruction than is available through ad hoc forms of digital health. Given the age-digital health interaction, it is possible that such skills may be more likely to develop at older ages, and with more life practice or, alternatively, that younger, more digitally-dependent patients are less able to develop self-talk and persistence skills. More research is needed to better understand these associations.
Higher digital health use also did not correlate with differences in number of ED or PCP visits. In other words, digital health use was not limited to people with high utilization of the healthcare system. Nor, despite its prediction of improved coping skills, did it correlate with decreased utilization of healthcare. Future research should prospectively examine the systems-level outcomes of digital health use among patients with chronic pain, controlling for known confounders of usage.
Finally, despite others’ work suggesting that women are more likely to seek out social support in the face of illness, female gender only weakly predicted improved self-talk and relaxation scores, and did not correlate at all with the other coping scales (30,31). However, our population was predominantly female, which may have decreased the power to detect differences.
Limitations
Due to the cross sectional study design, only a correlation between these factors can be noted, not a causation. Because the survey was administered via PatientsLikeMe, selection bias is present as these patients are already utilizing technology in conjunction with their healthcare. Additionally, these patients’ experiences may not be generalizable to the larger PLM patient population nor to the general chronic pain patient population. Finally, we did not assess the specific subtypes of digital health used (e.g., self-tracking, text-messaging, obtaining social support, etc.); nonetheless, the information about types used is a major contribution to the literature.
Conclusions
Digital health usage is common among a wide range of patients with chronic pain diagnoses. The usage of multiple forms of digital health (apps, social media, and online resources) is associated with improved chronic pain coping mechanisms. Future work should examine specific purposes and uses of digital health by this population, and develop theoretically-informed, evidence based support systems and interventions.
Acknowledgements
We would like to thank the patients of PatientsLikeMe.com who took part in our survey, Dr. Marcy Fitz-Randolph and Dr. Emil Chiauzzi for their guidance in survey development, and Ms. Sarah Pittman for her assistance with manuscript formatting.
Funding: Research reported in this presentation was funded through a Patient-Centered Outcomes Research Institute (PCORI) Award #IHS-1306-02960.
Footnote
Conflicts of Interest: The authors have no conflicts of interest to declare. Neither the funder nor PatientsLikeMe had any involvement in data analysis or manuscript authorship.
Disclaimer: The views in this work are solely the responsibility of the authors and do not necessarily represent the views of the Patient-Centered Outcomes Research Institute (PCORI), its Board of Governors or Methodology Committee.
References
- Hibbard JH, Greene J. What the evidence shows about patient activation: better health outcomes and care experiences; fewer data on costs. Health Aff (Millwood) 2013;32:207-14. [Crossref] [PubMed]
- Topol E. Creative Destruction of Medicine. New York: Basic Books; 2012.
- Albright K, Krantz MJ, Backlund Jarquín P, et al. Health promotion text messaging preferences and acceptability among the medically underserved. Health Promot Pract 2015;16:523-32. [Crossref] [PubMed]
- Anton SD, LeBlanc E, Allen HR, et al. Use of a computerized tracking system to monitor and provide feedback on dietary goals for calorie-restricted diets: the POUNDS LOST study. J Diabetes Sci Technol 2012;6:1216-25. [Crossref] [PubMed]
- Bates DW, Gawande AA. Improving safety with information technology. N Engl J Med 2003;348:2526-34. [Crossref] [PubMed]
- Patel S, Jacobus-Kantor L, Marshall L, et al. Mobilizing your medications: an automated medication reminder application for mobile phones and hypertension medication adherence in a high-risk urban population. J Diabetes Sci Technol 2013;7:630-9. [Crossref] [PubMed]
- Darkins A, Ryan P, Kobb R, et al. Care Coordination/Home Telehealth: the systematic implementation of health informatics, home telehealth, and disease management to support the care of veteran patients with chronic conditions. Telemed J E Health 2008;14:1118-26. [Crossref] [PubMed]
- Powell J, Inglis N, Ronnie J, et al. The characteristics and motivations of online health information seekers: cross-sectional survey and qualitative interview study. J Med Internet Res 2011;13:e20. [Crossref] [PubMed]
- Bergman AA, Matthias MS, Coffing JM, et al. Contrasting tensions between patients and PCPs in chronic pain management: a qualitative study. Pain Med 2013;14:1689-97. [Crossref] [PubMed]
- Holman H, Lorig K. Patient self-management: a key to effectiveness and efficiency in care of chronic disease. Public Health Rep 2004;119:239-43. [Crossref] [PubMed]
- Christiansen S, Gupta A. Can mobile technology improve treatment of chronic pain? Pain Med 2014;15:1434-5. [Crossref] [PubMed]
- Becker KL. Cyberhugs: creating a voice for chronic pain sufferers through technology. Cyberpsychol Behav Soc Netw 2013;16:123-6. [Crossref] [PubMed]
- Keogh E, Rosser BA, Eccleston C. e-Health and chronic pain management: current status and developments. Pain 2010;151:18-21. [Crossref] [PubMed]
- Rosser BA, McCullagh P, Davies R, et al. Technology-mediated therapy for chronic pain management: the challenges of adapting behavior change interventions for delivery with pervasive communication technology. Telemed J E Health 2011;17:211-6. [Crossref] [PubMed]
- Lalloo C, Jibb LA, Rivera J, et al. "There's a Pain App for That": review of patient-targeted smartphone applications for pain management. Clin J Pain 2015;31:557-63. [Crossref] [PubMed]
- Available online: https://www.patientslikeme.com/about
- National Center for Health Statistics. National Health Interview Survey, 2013. Public-use data file and documentation. (2013).http://www.cdc.gov/nchs/nhis/quest_data_related_1997_forward
- Jensen MP, Turner JA, Romano JM, et al. The Chronic Pain Coping Inventory: development and preliminary validation. Pain 1995;60:203-16. [Crossref] [PubMed]
- Romano JM, Jensen MP, Turner JA. The Chronic Pain Coping Inventory-42: reliability and validity. Pain 2003;104:65-73. [Crossref] [PubMed]
- Ranney ML, Choo EK, Wang Y, et al. Emergency department patients' preferences for technology-based behavioral interventions. Ann Emerg Med 2012;60:218-27.e48. [Crossref] [PubMed]
- Health Data Exploration Project. Personal Data for the Public Good: New Opportunities to Enrich Understanding of Individual and Population Health. University of California Irvine, University of California San Diego; 2014.
- Ranney ML, Suffoletto B. Extending our reach: use of mHealth to support patients after emergency care. Ann Emerg Med 2014;63:755-6. [Crossref] [PubMed]
- Hart T, Ahlers-Schmidt CR, Chesser A, et al. Physician impressions of using text message technology to increase vaccination compliance. Telemed J E Health 2011;17:427-30. [Crossref] [PubMed]
- Gagnon MP, Ngangue P, Payne-Gagnon J, et al. m-Health adoption by healthcare professionals: a systematic review. J Am Med Inform Assoc 2016;23:212-20. [Crossref] [PubMed]
- Yarbrough AK, Smith TB. Technology acceptance among physicians: a new take on TAM. Med Care Res Rev 2007;64:650-72. [Crossref] [PubMed]
- Campbell O. Designing for the elderly: ways older people use digital technology differently. Smashing Magazine. February 5th, 2015.
- Haederle M. Technology fear stops older adults from logging on. AARP Bulletin. 2011.
- Miskelly FG. Assistive technology in elderly care. Age Ageing 2001;30:455-458. [Crossref] [PubMed]
- Ancker JS, Witteman HO, Hafeez B, et al. "You get reminded you're a sick person": personal data tracking and patients with multiple chronic conditions. J Med Internet Res 2015;17:e202. [Crossref] [PubMed]
- Awasthi P, Mishra RC. Role of coping strategies and social support in perceived illness consequences and controllability among diabetic women. Psychology Developing Societies 2007;19:179-97. [Crossref]
- Weinert C. Social support in cyberspace for women with chronic illness. Rehabil Nurs 2000;25:129-35. [Crossref]
Cite this article as: Ranney ML, Duarte C, Baird J, Patry EJ, Green TC. Correlation of digital health use and chronic pain coping strategies. mHealth 2016;2:35.