Drawing open the curtain on home-based interventions
Introduction
Providing facility-based care to the billions of people living in Low- and Middle-Income Countries (LMICs) is a challenge due to the multitude of barriers people face in accessing these sites. Unaffordable transportation costs, limited child care options, poor health and inconsistent staffing and services of facilities are just some of the many reasons facility-based primary health care interventions struggle to recruit and retain patients in efficacious programs (1). Home-based interventions have been shown to be a viable alternative across a broad range of health initiatives including infectious disease (e.g., HIV screening), mental health (e.g., postpartum depression) and non-communicable disease risk reduction and education (e.g., obesity and nutrition) interventions (2-4). One of their key weaknesses is lack of cost-effectiveness. Multiple follow-up visits to the home are required to deliver the intervention and to ensure that uptake and behavioural change are taking place. A second limitation of home-based interventions is that they are typically one-size-fits-all. Lay health workers are trained in the intervention and the sessions and manuals are routinized to simplify deliver and to increase the likelihood of intervention fidelity. We believe a solution to these challenges is available in the use of passive sensor data to provide robust, evidence-based feedback on what happens in the home after the health worker walks out the door.
Passive sensing data
Many terms exist to describe the phenomena of using mobile phones’ sensors and other Internet of Things (IoT) devices embedded in the environment to passively collect information about people’s behaviour. “digital data exhaust”, “digital phenotyping” and “IoT for healthcare” are all terms currently used to describe this idea. In LMIC these digital sensors and the machine learning algorithms required to process large volumes of sensor data are needed to actively monitor individuals and families receiving health interventions at home. Key questions that sensor data can address are why the health problem is occurring, who else in the family is involved in facilitating or impeding care and recovery, when changes in health and behaviour are happening across both short (minute to minute) and long (month to month) timescales, and what aspects of the intervention are being implemented.
Why is there a health problem for this individual?
Obesity is a multifaceted health risk including individual level factors such as caloric intake and exercise patterns to family level factors such as norms around portion size and the lack of income to purchase nutrient-dense food to societal factors such as a country undergoing an obesogenic transition. Considering the individual for this example, a traditional approach would be to conduct a baseline survey about activity and diet. These data are known to often suffer from both under and over reporting bias and may not give an accurate representation of the problem for this particular individual (5). An alternative approach might be to deploy a suite of home sensors to monitor physical activity (not only steps but time sitting, standing and running), air quality (to establish frequency and timing of food preparation), sleep and snoring patterns (with reduced sleep being known to have an association with weight gain) and photographs from a lapel worn camera to capture food eaten during the day. Figure 1 depicts the images taken from such a camera carried by a 5-year-old child in rural South Africa, which gives an interesting insight into the types of foods being consumed, such as high-calorie beverages. Rather than the intervention focusing on moving from a starch rich diet, typical in this community, the intervention could instead focus on reducing fast food and sugary drinks.
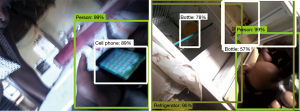
Who else needs to be included in the intervention?
Mobile phone call logs provided a valuable source of data about people and their social network. Harvesting such data for analysis is a subject of privacy concern and requires ethical approval and compliance with legal frameworks such as the General Data Protection Regulation (EU GDPR). Comulada provides an example of how call log data can be ethically collected in order to facilitate health intervention (6). The egocentric networks of 12 gay men (n=44 total participants) were enumerated by automated collection of log data from the men’s Android smartphones. These call logs were able to predict primary partner as confirmed via self-report. Infectious disease transmission and non-communicable disease risk factors occur between people and within relationships and family. Proximity (inferred from Bluetooth advertising packets), audio recordings of verbal interactions and time spent together could all be used to supplement call logs to more accurately enumerate these relationships and social networks in order to ensure that intervention delivery is targeted towards the correct set of individuals. These suggestions and approaches clearly raise major questions about privacy, confidentiality and anonymity. While techniques and approaches are being developed, for example to extract only a subset of non-identifying features from audio data, other research seeks to reconstruct these de-identified datasets. Using a generative copula-based method Rocher, Hendrickx & de Montjoye found that it was possible to re-identified 99.98% of people in any dataset using only 15 demographic variables (7).
When is the best time to prompt or trigger action?
Theoretical models of behaviour change often include the concept of a trigger provided at just the right time with the expectation that the nudge will illicit the behaviour of a monitored individual (8). The field of Behaviour Design suggests that the timing of these triggers is crucial (9). A promising application of triggers is in Behavioural Activation interventions designed to reduce postpartum depression. We are currently exploring this approach with depressed mothers (“Sensing technology to personalize adolescent maternal depression treatment in low resource settings”, Project STANDSTRONG) in rural Nepal (10). We used qualitative anthropological procedures to select passive sensing technology that would be culturally acceptable and contextually feasible (11). The study provides depressed mothers with a set of actions and activities. They are given a small positive reward if they follow the suggested action points. Using information about a mother’s daily routine, established by monitoring GPS and accelerometer data sources, triggers for these mood enhancing activities will be targeted to arrive on the mother’s phone at a time she is most likely to be able to act on them. Depressed mothers also often have a very disruptive and irregular daily routine. Using passive sensors such as sleep sensors, light sensor readings, and proximity beacons to track time with her child, we provide information about this daily routine to a community health worker who is able to use the information in counselling sessions to see how a more regular daily pattern could be established, and thus personalize the intervention.
What are individuals and families doing with the information after they receive the intervention?
Insulin control is the primary intervention for diabetes. The importance of carefully monitoring insulin levels and taking care about the types of food that get eaten will be stressed by all health workers counselling patients newly diagnosed with diabetes. Once the health worker leaves the home, however, no feedback loop exists to monitor adherence in order to provide additional support if required. Long-term indicators such as haemoglobin A1c fail to provide quotidian information on dietary and exercise behaviours. The quality of care, cost-effectiveness of the intervention and arguably improved outcomes could be achieved if there was an easy way to passively monitor these factors. An example of such an approach is provided by Gu et al. (12) (2017) SugarMate is a non-invasive smartphone-based blood glucose inference system. Leveraging smartphone sensors, SugarMate automatically measures physical activity and sleep quality along with insulin and food intake collected through an ecological momentary assessment app. These data, when passed through a Multi-division Deep Dynamic Recurrent Neural Network (MdRNN), yield an average blood glucose level prediction that has an accuracy of 82.14%.
What are some of the current technical challenges and solutions to the collection of remote sensing data?
Data collection, transmission, and processing of observations are crucial aspects of all the data systems presented. Although the IoT is a broad concept that includes diverse technologies, such as robots, drones, cars and software agents, it is mostly dominated by low battery-powered devices. Sending raw sensor data can lead to communication overhead and high energy consumption. As a remedy, the utilisation of time-series data compression approaches can help in dealing with large volumes of (near) real-time data. Nevertheless, there is a trade-off between computational cost versus communication overhead (13). While it is desirable to minimise the computational cost, power consumption in communication is usually significantly higher than the computational cost (14,15). Therefore, it is important to use lightweight, adaptive, yet effective time series analysis approaches in stream processing. Once time-series data is compressed, a change or event detection technique needs to be employed for sensors to send data only when there is a new event in the environment.
Furthermore, IoT Healthcare applications have to handle heterogeneous data streams and data sources, which involve a large number of input formats, while it needs to produce a unified format as output for the decision-making systems. There has been a significant amount of work on this problem. The Semantic Sensor Network (SSN) ontology (16) is one of the most significant efforts in the development of an information model for sensory data. The SSN ontology provides a vocabulary for describing concepts such as sensors, outputs, observation value and feature of interests. It has recently been revised by including a lightweight SOSA ontology (Sensor, Observation, Sample, and Actuator). Although the SSN ontology defines a high-level scheme of sensor systems, it does not include representation of observation and measurement data. Other notable initiatives include the IoT-A model (17) and IoT.est semantic representations (18), that describe how to enhance the utilisation and representation of domain knowledge in sensor networks, with the IoT-A model providing an architectural base for further IoT projects, and IoT.est enhancing the IoT-A model with some service and test concepts.
Conclusions
A key consideration of passive sensing data systems is their ethical use. Data can be invasive and encroach on people’s privacy, and it is crucial to assure data security of information collected from the home. Techniques to transform visual and audio information that do not allow easy reconstruction of personal identifying information are required. A second ethical consideration is how best to explain these sensors, and the data they collect, to participants that may not have a strong technical grasp of what is being proposed. Some work has started to address these concerns, but there is a definite need for more effort to be put into making these tools safe, ethical and as non-invasive as possible. Although the field is still young and maturing, there is enough evidence to suggest that more rigorous experimental designs are required to establish how to most effectively harness passive sensing data as a new tool in the interventionists tool belt. In order to avoid the overextended “pilotitis” phase that the mHealth field went through during its early years, it is suggested that those wanting to engage with this new tool focus early and sharply on establishing cost-effectiveness and health outcomes through rigorous randomised control trials. Passive sensing data collection holds the potential to teach us a tremendous amount about people’s lives and health. We will need study designs that clearly capture the why, what, who, and when of home-based interventions while addressing the ethics of this rapidly evolving arena.
Acknowledgments
Funding: This research was partially supported by the National Institute of Mental Health (No. R21MH106351 and P30MH058107) and Grand Challenges Exploration (No. OPP1189927).
Footnote
Conflicts of Interest: All authors have completed the ICMJE uniform disclosure form (available at http://dx.doi.org/10.21037/mhealth.2020.01.02). The authors have no conflicts of interest to declare.
Ethical Statement: The authors are accountable for all aspects of the work in ensuring that questions related to the accuracy or integrity of any part of the work are appropriately investigated and resolved.
Open Access Statement: This is an Open Access article distributed in accordance with the Creative Commons Attribution-NonCommercial-NoDerivs 4.0 International License (CC BY-NC-ND 4.0), which permits the non-commercial replication and distribution of the article with the strict proviso that no changes or edits are made and the original work is properly cited (including links to both the formal publication through the relevant DOI and the license). See: https://creativecommons.org/licenses/by-nc-nd/4.0/.
References
- Bohren MA, Hunter EC, Munthe-Kaas HM, et al. Facilitators and barriers to facility-based delivery in low- and middle-income countries: a qualitative evidence synthesis. Reprod Health 2014;11:71. [Crossref] [PubMed]
- Kohrt B, Asher L, Bhardwaj A, et al. The Role of Communities in Mental Health Care in Low- and Middle-Income Countries: A Meta-Review of Components and Competencies. Int J Environ Res Public Health 2018;15:1279. [Crossref] [PubMed]
- Gaziano TA, Abrahams-Gessel S, Denman CA, et al. An assessment of community health workers’ ability to screen for cardiovascular disease risk with a simple, non-invasive risk assessment instrument in Bangladesh, Guatemala, Mexico, and South Africa: an observational study. Lancet Glob Heal 2015;3:e556-63. [Crossref] [PubMed]
- Sabapathy K, Van den Bergh R, Fidler S, et al. Uptake of home-based voluntary HIV testing in sub-Saharan Africa: a systematic review and meta-analysis. PLoS Med 2012;9:e1001351 [Crossref] [PubMed]
- Grandjean AC. Dietary intake data collection: challenges and limitations. Nutr Rev 2012;70:S101-4. [Crossref] [PubMed]
- Comulada WS. Mobile Phone Assessment in Egocentric Networks: A Pilot Study on Gay Men and Their Peers. Connect (Tor) 2014;34:43-51. [Crossref] [PubMed]
- Rocher L, Hendrickx JM, de Montjoye YA. Estimating the success of re-identifications in incomplete datasets using generative models. Nat Commun 2019;10:3069. [Crossref] [PubMed]
- Nahum-Shani I, Smith SN, Spring BJ, et al. Just-in-Time Adaptive Interventions (JITAIs) in Mobile Health: Key Components and Design Principles for Ongoing Health Behavior Support. Ann Behav Med 2018;52:446-62. [Crossref] [PubMed]
- Fogg BJ. Persuasive technology. Ubiquity 2002;2. [Crossref]
- Poudyal A, van Heerden A, Hagaman A, et al. Wearable Digital Sensors to Identify Risks of Postpartum Depression and Personalize Psychological Treatment for Adolescent Mothers: Protocol for a Mixed Methods Exploratory Study in Rural Nepal. JMIR Res Protoc 2019;8:e14734 Erratum in: Correction: Wearable Digital Sensors to Identify Risks of Postpartum Depression and Personalize Psychological Treatment for Adolescent Mothers: Protocol for a Mixed Methods Exploratory Study in Rural Nepal [JMIR Res Protoc. 2019]. [Crossref] [PubMed]
- Kohrt BA, Rai S, Vilakazi K, et al. Procedures to Select Digital Sensing Technologies for Passive Data Collection With Children and Their Caregivers: Qualitative Cultural Assessment in South Africa and Nepal. JMIR Pediatr Parent 2019;2:e12366 [Crossref] [PubMed]
- Gu W, Zhou Y, Zhou Z, et al. SugarMate: Non-intrusive blood glucose monitoring with smartphones. Proceedings of the ACM on Interactive, Mobile, Wearable and Ubiquitous Technologies, 2017:1-27.
- Kolozali Ş, Puschmann D, Bermudez-Edo M, et al. On the Effect of Adaptive and Nonadaptive Analysis of Time-Series Sensory Data. IEEE Internet Things J 2016;3:1084-98. [Crossref]
- Anastasi G, Falchi A, Passarella A, et al. Performance measurements of motes sensor networks. In: Proceedings of the 7th ACM International Symposium on Modeling, Analysis and Simulation of Wireless and Mobile Systems. MSWiM ’04. New York, New York, USA: ACM Press, 2004:174-81.
- Chong SK, Gaber MM, Krishnaswamy S, et al. Energy-Aware Data Processing Techniques for Wireless Sensor Networks: A Review. In: Hameurlain A, Küng J, Wagner R. editors. Transactions on Large-Scale Data- and Knowledge-Centered Systems III. Berlin, Heidelberg: Springer, 2011:117-37.
- Compton M, Barnaghi P, Bermudez L, et al. The SSN ontology of the W3C semantic sensor network incubator group. Journal of Web Semantics 2012;17:25-32. [Crossref]
- De S, Elsaleh T, Barnaghi P, et al. An Internet of Things Platform for Real-World and Digital Objects. Scalable Computing Practice and Experience 2012;13:45-57.
- Wang W, De S, Toenjes R, et al. A Comprehensive Ontology for Knowledge Representation in the Internet of Things. In: 2012 IEEE 11th International Conference on Trust, Security and Privacy in Computing and Communications. 25-27 June 2012; Liverpool, UK. IEEE, 2012:1793-8.
Cite this article as: Van Heerden A, Comulada WS, Kolozali Ş, Kohrt B. Drawing open the curtain on home-based interventions. mHealth 2021;7:18.