Feasibility of community health workers using a clinical decision support system to screen and monitor non-communicable diseases in resource-poor settings: study protocol
Introduction
Globally, the burden of disease has dramatically shifted from communicable, maternal, perinatal, and nutritional causes towards non-communicable diseases (NCDs) (1). NCDs now constitute a significant threat to global health, being responsible for approximately 72% of all deaths in 2016 (1). According to the World Health Organization (WHO), NCDs result in an estimated 40 million deaths per year (2). More than three-quarters of these deaths occur in low- and middle-income countries (LMICs) (3). By 2020, it is predicted that NCDs will be responsible for causing seven out of every ten deaths in LMICs (4). Similar to many other LMICs, in India, diabetes, cardiovascular diseases (CVDs), and their complications are a growing problem. India is also on the way to becoming the global ‘hypertension capital’ (5) with ~207 million people with hypertension (6). CVDs are the leading cause of death globally, accounting for approximately 17.6 million deaths annually. Globally, CVDs increased in incidence by 14.5% between 2006 and 2016 (7). Hypertension is the largest contributor to the global burden of disease (8) and the number of people with hypertension has almost doubled from 442 million in 1990 to 874 million in 2015 (9). Approximately 75% of deaths due to complications from high blood pressure occur in LMICs (9). Diabetes is also a major problem, being present in approximately 370 million people globally. This number is expected to exceed 550 million by 2030, with 80% of this disease burden being in LMICs (10). Anemia is also one of the independent risk factors for CVDs and renal disease in patients with hypertension (11). For example, the prevalence of normocytic anemia is significantly higher in hypertensive patients (12). With most LMICs undergoing an epidemiological transition (13), the lifestyle-related risk factors that contribute to CVDs and their macrovascular and microvascular complications, such as smoking, unhealthy diet, and physical inactivity are becoming more prevalent (14).
Data from high-income countries provide evidence that an integrated primary care-based preventive program has the potential to reduce lifestyle-related risk factors contributing to NCDs (15). However, there are many challenges for such approaches in LMICs. These include shortages of qualified practitioners, lack of competencies among the health workforce, lack of proper referral and poor infrastructure of health centers and hospitals (16). Together, these factors result in suboptimal functioning of the primary healthcare system. Hence, there is a need to address these issues by increasing the workforce and increasing access to routine health care. A potential way to do that is by sharing tasks between non-physician health workers [i.e., nurses, paramedics, and community health workers (CHWs)] and doctors (17).
There is evidence that CHWs have the capacity to improve antenatal, perinatal, and postpartum service utilization by early recognition and referral of complicated cases (18,19). A recent report from the WHO provides evidence that CHWs, with proper training, can successfully capture data and deliver NCD-related services (20). CHWs have also been shown to be effective in tobacco cessation, and control of hypertension and diabetes, in some LMICs (21). Therefore, task sharing can be an effective strategy whereby identification and screening of cases of NCD are carried out by CHWs. Following identification, complicated cases, such as patients with high blood pressure or high blood glucose, can be referred to a medical doctor for further evaluation and management.
Handheld devices such as smartphones and tablets equipped with a clinical decision support system (CDSS) can be used to support and develop the capacity of CHWs when they are on their own in the community. CDSSs are computer-based programs that are used to analyze data within electronic health records to provide health workers with patient-specific assessments or recommendations to aid clinical decision making (18). There are two main types of CDSS (knowledge base or without knowledge base) to be used in the health care facility (22). CDSSs with knowledge base use patient data to display the results, on the other hand, CDSSs without a knowledge base, use a machine learning to analyze clinical data (22).
CDSSs have been used to improve drug prescribing practices (23,24), reduce serious medication errors (25,26), enhance the delivery of preventive care (27,28), and improve adherence to standard care practices (29,30). The CDSS is a recent strategy to support health care providers in delivering a variety of interventions. They have the potential to facilitate the prevention of NCDs in various ways. For example, these systems can be utilized to remind providers to screen risk factors, and flag cases of hypertension and diabetes, with or without other co-morbidities. CDSSs can also be designed to provide details of treatment protocols, prompt questions about adherence to medication, and provide lifestyle modification interventions for healthy behavior. Hence, there is scope for these devices to support CHWs, supporting them to screen people with risk factors for NCDs and help them to determine probable diagnoses. With proper training, CHWs, supported by a CDSS, have the capacity to identify and refer urgent cases, thereby reducing the incidence of acute complications of CVDs in the community (i.e., stroke, heart attack, and renal failure). If CHWs can perform triage (screen, classify, and refer), it will help to reduce the workload of the physician working at the first referral point of primary care. To do so, CHWs need correct resources, training and support.
The purpose of this study is to test the feasibility of a CDSS and an educational training program. This CDSS is aimed at assisting CHWs in resource-poor settings to identify, diagnose, refer and monitor people with hypertension and co-morbidities (i.e., anemia, pre-diabetes, diabetes, hyperlipidemia or CVDs). We aim to determine whether the CDSS is user-friendly, easy-to-comprehend, easy-to-deliver, workflow-oriented, and comprehensive. We will also determine the effectiveness of a training program for improving the knowledge and skills of ASHAs.
We present the article in accordance with the SPIRIT reporting checklist (available at http://dx.doi.org/10.21037/mhealth-19-258).
Methods
Study design and location
We will conduct operational research to assess the feasibility (usability and acceptability) of a CDSS and undertake a prospective educational trial to determine the effectiveness of a training program. The study will be conducted near Chamba, in the Tehri district of Uttarakhand in India. The Tehri district is designated as a middle hills region and has a population of 604,608 people. The vast majority of the District’s population (approximately 89%) live in small villages. The difficult terrain in the Tehri District contributes to the difficulties in accessing health care. We will utilize the existing system of Accredited Social Health Activists (ASHAs), who are CHWs, to build and strengthen their capacity to prevent and control hypertension and diabetes in the community. We will train these ASHAs to operate the CDSS and deliver a lifestyle intervention (31,32) to manage NCDs. First, we will use a semi-structured questionnaire to determine details about the acceptance of using the app, satisfaction with the CDSS, perceived barriers, ideas for improvement, and willingness to use the CDSS. We will also test the usability of this CDSS for the identification of people with hypertension, with or without co-morbidities, by ASHAs and their supervisors. Second, to develop capacity within the ASHA workforce, ASHAs will be taught about NCDs, so they can motivate people to adhere to healthy activities and self-manage their NCDs. Ethical permission to conduct the study has been approved by the ethics committee of the Garhwal Community Development and Welfare Society India and the Monash University Human Research Ethics Committee. This study will be conducted in accordance with the Declaration of Helsinki (as revised in 2013) and the Harmonized Tripartite Guideline for Good Clinical Practice from the International Conference on Harmonization; the Indian Council of Medical Research (ICMR); Guidelines for Biomedical Research in Human Subjects 2006, with any subsequent amendments; and all applicable laws of the Republic of India. All participating ASHAs and participants will sign an informed consent form which will be stored safely.
Conduct of the study/implementation plan of the study
This mixed-method study involves both qualitative and quantitative approaches. The study will be conducted in two phases to test: (I) feasibility of the CDSS itself, and (II) feasibility of utilizing the app to develop capacity within the ASHA workforce (Figure 1). This study includes free screening, the use of culturally appropriate education material, provision of affordable long-term management, and referral of critical cases to a doctor or health center.
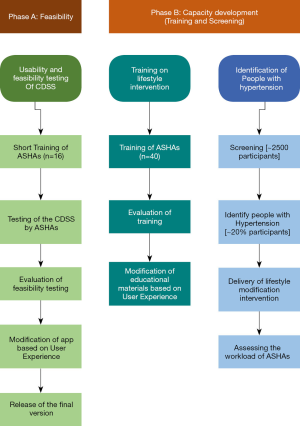
Phase A: feasibility (acceptability and usability) testing of the Arogya Sahyog
Planned activities
In partnership with the Monash Institute of Information Technology, we have developed a beta version of Arogya Sahyog. The app is programmed to an android operating system, as these devices are widely available and affordable in LMICs, particularly in India. This Arogya Sahyog app not only works as a CDSS but also helps researchers in collecting accurate data. This app, installed on a tablet device, is designed to help ASHAs screen, provide lifestyle advice, and refer sick people to primary care physicians (Figure 2). A screening and management algorithm has been developed for the app based on the Indian Hypertension Guidelines (33). We have prepared a user manual illustrating the use and functions of Arogya Sahyog. These instructions will also help ASHAs to navigate the app. We will conduct a 5-day training program, together with practical sessions, so that ASHAs can learn how to operate the app on a tablet device. After training the ASHAs, we will test the stable version of the app [Arogya Sahyog] in a real-life setting.
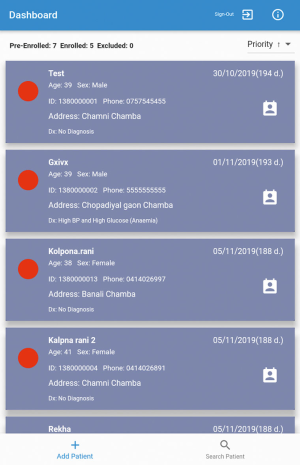
Evaluation
The usability of the app will be assessed using the system usability scale (SUS) (34). We will use a semi-structured questionnaire to determine details about the acceptance of using the app, satisfaction with the CDSS, perceived barriers, ideas for improvement, and willingness to use the CDSS. ASHAs will also be asked about the usability of the educational materials and the challenges they encountered in understanding the content during the training sessions. Using direct observation by their supervisor, we will also assess their ability to screen and manage four to five participants (case scenarios) using the CDSS. Some ASHAs will role-play a case-scenario of a ‘pretend’ participant with set answers while being interviewed by another ASHA. They will then switch roles.
Software refinement
If the SUS score or feedback from the participants indicate the need to improve the beta version of the CDSS, a set of criteria for software revision will be defined. The software version will be modified accordingly.
Phase B: (I) evaluating the effectiveness of the training program to increase knowledge of ASHAs
Planned activities
Training manuals have been developed to teach ASHAs how to use a digital blood pressure machine and other point-of-care devices. The devices include those for measuring blood glucose, glycated hemoglobin, and blood lipids, as well as height, weight, and waist and hip circumference. The training package includes details of screening, basic management (lifestyle modification strategies), and materials to help them educate patients. Training will last approximately 5–6 days, with about 40 ASHAs participating in the training. The training materials have been designed to include all aspects of monitoring targets for BP and other relevant risk factors that have been incorporated in the app. They also include details on how to advise people with hypertension to adhere to a healthy lifestyle and how to provide one-on-one self-management support. This training package has been modified from the WHO Package of Essential Non-communicable Disease Interventions (PEN) Guideline, the ‘Controlling Hypertension in Rural India (CHIRI)’ study (35), and existing training packages for ASHAs (36).
Evaluation and refinement
We will test ASHAs’ knowledge and skills about NCDs before and after training. The test consists of multiple-choice and true/false type questions about hypertension, its risk factors, complications, and techniques for adopting a healthy lifestyle. We will compare the knowledge of 20 ASHAs who take part in this study with that of another group of ASHAs (~20 ASHAs) who have not participated in the training. We will evaluate whether this training has improved the knowledge and skills of the ASHAs who will be trained. We will also interview four to five ASHAs and their supervisors to understand the usability of the educational materials. We will use an evaluation form (37) to collect their feedback on the training materials. Based on feedback from the trainees and participants, we will further refine the training materials.
Phase B: (II) assessing the capabilities of ASHAs to identify people with NCDs
Planned activities
At the time that ASHAs are trained in NCDs, use of blood pressure devices and point of care devices, we will also teach them how to use the CDSS. The CDSS is incorporated in the app on the tablet device, and ASHAs will receive hands-on training on the use of the app and tablet device to identify people with high blood pressure with or without co-morbidities. Following the completion of the training program and assessment of knowledge and skills, ASHAs will use the tablet device to provide screening services at the household level.
On the first day, the ASHA will go to the household and identify people aged at least 30 years. She will assess each person using the community-based assessment checklist (CBAC) as provided by the Ministry of Health and Family Welfare (36), and then inform participants about their risk assessment score (Figure 3). The app enables automatic calculation of the risk score and provides instructions according to the Indian government guidelines for the CBAC. When participants have a CBAC of more than 4, ASHAs will advise them to attend the NCD day in a sub-center.
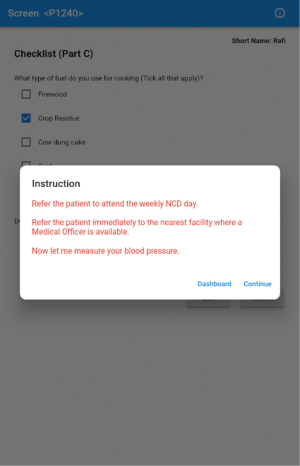
Irrespective of CBAC scores, BP will be measured for each participant three times. The minimum gap will be three minutes between measurements. If the average blood pressure is ≥140/90 mmHg for the second and third measurements or participants provide a history of taking antihypertensive medication, ASHAs will initially include the participants (Figure 4) and schedule them for a follow-up visit. Then, the ASHAs will measure hemoglobin and blood glucose using point of care devices.
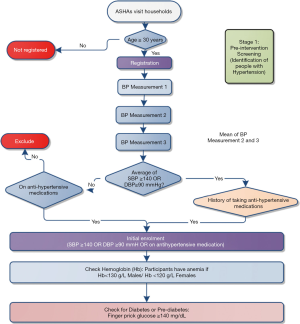
ASHAs will be required to enter the results of all tests from point-of-care devices in the CDSS. Based on the average blood pressure and the levels of blood glucose and hemoglobin, the CDSS enables the computation of diagnosis based on the in-built algorithm. This algorithm has been designed according to the standard guideline (36). For example, if the blood pressure, and blood glucose of the participant are above the acceptable range and the hemoglobin level of the participant is below the acceptable range, the CDSS will show ‘High BP, High Glucose and Anemia’ as a probable diagnosis in the pop-up screen.
Stage 2: For those participants who have initially been included, ASHAs will re-visit the household after seven days to re-measure BP; three times for each participant. If the average BP for the second and third assessments is ≥140/90 mmHg, or the participant is taking blood pressure-lowering medications, the participant will be considered as hypertensive and so included for ongoing monitoring (Figure 5). For participants who have been assessed as having a diagnosis of hypertension, ASHAs will then use point of care devices to measure blood glucose and glycated hemoglobin (HbA1c) for diagnosis of diabetes and lipid profile for diagnosis of hyperlipidemia. When these details are entered onto the app, the CDSS will help ASHAs to identify critical patients based on the algorithm. The CDSS will also provide instruction to ASHAs to urgently refer those critical patients to the nearest health center. ASHAs will also measure anthropometry and collect information on demographics, socioeconomic status, health literacy, use of health care services and quality of life.
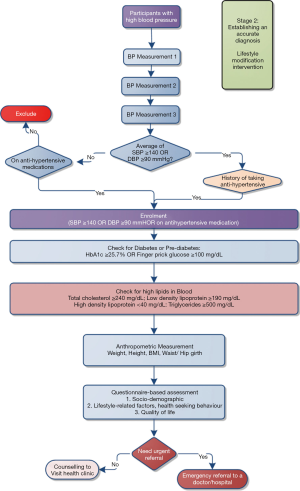
Patients with hypertension showing the following signs and or symptoms will be referred immediately:
- The appearance of any new/severe chest pain;
- Symptoms/signs of transient ischemic attack/stroke (sudden weakness/trouble seeing/confusion/trouble walking/severe headache);
- Symptoms/signs of heart failure (shortness of breath, difficulty breathing);
- Blood pressure >180/110 mmHg;
- Plasma glucose ≥18 mmol/L;
- Foot ulcers.
If the patient does not need an urgent referral, ASHAs will deliver a lifestyle intervention to the participants as prompted by the CDSS. All the educational materials designed for each lifestyle intervention are provided within the CDSS. In addition, the built-in dashboard in the app will visualize graphs to show the participant’s status (and change over time). ASHAs will be able to explore the overall condition of a participant using this built-in dashboard. In these critical cases, ASHAs will also advise each participant to visit a medical doctor for further evaluation and management of hypertension and any other co-morbidities that have been identified.
Evaluation
After successful completion of training, each ASHA will screen and counsel approximately 4–5 participants under the direct observation of a designated supervisor. The supervisor will be highly trained in use of this CDSS device and act as a gold standard. In doing so, we will recruit a supervisor who is educated with a graduate degree and experienced in working with ASHAs. We will organize special hands-on training on the use of the app for the supervisor.
The supervisor will assess the performance of ASHAs by marking off their capability using a pre-designed checklist. The checklist will include details such as the tasks delivered, use of resources, time management, and problem-solving skills of the ASHA. The supervisor will also assess the time needed by the ASHA to screen, including measuring blood pressure and undertaking other procedures. Additionally, the device will be used to measure the time required to conduct blood pressure measurements, biochemical tests, and anthropometry. When the ASHA clicks a particular button, the app will start measuring the time, and this continues until all the measurements have been taken and recorded. Furthermore, a supervisor will be assigned to assess the performance of ASHAs.
Statistical considerations
Phase A: feasibility (acceptability and usability) testing of the Arogya Sahyog
Sample size
Approximately 16 ASHAs will be invited to test the beta version of Arogya Sahyog. As this is a feasibility study, the primary outcome of the feasibility study is the usability of Arogya Sahyog. Therefore, a formal sample size calculation is not relevant (38).
Data analysis
The usability of the application will be analyzed by determining the SUS score. The SUS score ranges from 0 to 40. Each score will be multiplied by 2.5 to convert the result to a percentage (34,39). We will calculate the average of single-item scores and the overall score. According to the literature, an overall SUS score ≥70% denotes good usability, while a score ≤50% indicates a considerable need for improvement (39). This feasibility testing will be considered successful when a SUS score of ≥70% is reached. We will use kappa statistics to determine the agreement between assessments recorded by ASHAs with the gold-standard answers of the case-scenarios. Descriptive statistics will be used to report the acceptance, satisfaction, and fidelity of the Arogya Sahyog application.
Phase B: (I) evaluating the effectiveness of the training to increase knowledge of ASHAs
Sample size
We will recruit two groups of ASHAs, one of which will receive training about NCDs using specifically designed educational materials. The second group of ASHAs will not receive this training. Our outcome of interest is to determine the change in the knowledge and skills of ASHAs to provide NCD services. Using a change in knowledge of 20 points more in the group provided training than those not provided training, alpha 0.05, and a sample size of 40 ASHAs (20 trained and 20 untrained) provides us with 80% power to detect this difference between groups. About 4–5 ASHAs will also be asked about the usability of the educational materials and what challenges they have encountered as they tried to understand the contents during the training session.
Data analysis
The demographic characteristics of ASHAs will be summarized using descriptive statistics. Quantitative data regarding knowledge and skills will be assessed using Student’s two-tailed paired t-test for comparing scores within groups. An independent two-sample t-test will be conducted to evaluate between-group differences in scores (trained versus non-trained group). We will calculate the score for the performance of each ASHA using the supervisor’s monitoring sheets (hard copies). We will use Kirkpatrick’s evaluation framework to evaluate the feedback of ASHAs and their supervisors about the training and educational materials (40).
Phase B: (II) Assessing the capability of ASHAs to identify people with NCDs
Sample size
Each ASHA will perform screening and counseling of 3–4 participants under the direct observation of a supervisor. The supervisor will assess the performance of ASHAs using a checklist of tasks, use of educational resources, and time management. The total number of structured observations of 40 ASHAs will, therefore, be approximately 120–160.
Each ASHA will then screen approximately 60–65 participants aged ≥30 years. We expect that approximately 20% of those screened will be positive for hypertension (41) so that each ASHA will identify about 12–13 people with hypertension (a total of approximately 500 people with hypertension). ASHAs will systematically visit selected households in the village, revisiting households when eligible members are not available during the initial visit.
Data analysis
Participant’s background characteristics will be reported using descriptive statistics. The estimates will be stratified by the provider-related information: age, sex, educational status, experience, etc. The difference in proportions across different stratifying variables will be assessed using Pearson’s χ2 test. We will use the Shapiro-Wilk test to assess normality. If the distribution of the time taken to undertake assessments does not violate normality, we will use means, standard deviations, and t-tests to determine differences between groups. However, if the distribution fails the test for normality, we will use medians, interquartile ranges and Wilcoxon Mann–Whitney tests to assess these differences. The level of agreement between ASHAs and supervisors will be reported using kappa statistics. All analyses will be conducted using Stata 14.0 (Stata Corporation, College Station, TX, USA) (19).
Quality control and data management of the overall study
The data will be collected using 7-inch tablet devices (37). The use of tablets is anticipated to minimize error rates on data entry since the database will be built with data field restrictions to ensure only valid answers are acceptable. Data will be uploaded to a central server during periods of functional internet connectivity. We will use a No-SQL server for data upload. Stata 14.0 will be used for data cleaning, management, and analysis. The supervisor will use a paper-based checklist to evaluate the performance of the ASHAs. The collected data will be entered into an excel spreadsheet specially designed for this study. We will also build a web-based dashboard to monitor the progress of data collection.
Discussion
A challenge for CHWs is to accurately deliver non-pharmacological approaches as a means to prevent CVDs (e.g., advice on healthy behavior and lifestyle modification). This approach also includes motivating people in the community and providing them with adequate support to adhere to healthy lifestyles. Delivering appropriate health education based on each patient’s needs can be a useful way for CHWs to promote healthy behaviors. However, three areas of competency (42) are needed for CHWs: (I) a basic understanding of hypertension and diabetes; (II) basic counseling competencies, including listening and communication skills, especially empathic listening; and (III) advocacy, to deliver health education. Each of these elements is included in the training package.
This study will give us the insight to determine whether ASHAs can use Arogya Sahyog to screen and manage NCDs. We will assess whether training of ASHAs increases their knowledge and skills to deliver a lifestyle modification intervention for people with hypertension with other co-morbidities (pre-diabetes, diabetes, anemia, hyperlipidemia) using a tablet device. Analyzing their workload will give us an understanding of the potential for future engagement of ASHAs for managing NCDs within the context of their assigned job in the field of maternal and child health. After determining the feasibility, and after final approval of the Arogya Sahyog application and training materials, we aim to evaluate the effectiveness of the CDSS and training materials in the management of NCDs in a large multi-center cluster randomized trial.
Acknowledgments
The authors are grateful to Farid Raisi, Tian Goh, Lana Coleman, Mulugeta Birhanu, Rajshree Thapa, Hem Prakash and Manoj Kumar, for their support to improve the manuscript.
Funding: This work is supported by intra-mural funding from Monash University, Australia. SBZ received a scholarship from the Australian Government research training program (RTP) in support of his academic career. This funding source had no role in the design of this study and will not have any role during its execution, analyses, interpretation of the data, or decision to submit results.
Footnote
Provenance and Peer Review: This article was commissioned by the Guest Editors (Shariful Islam and Ralph Maddison) for the series “Digital Health for Cardiovascular Disease” published in mHealth. The article was sent for external peer review organized by the Guest Editors and the editorial office.
Reporting Checklist: The authors have completed the SPIRIT reporting checklist. Available at http://dx.doi.org/10.21037/mhealth-19-258
Conflicts of Interest: All authors have completed the ICMJE uniform disclosure form (available at http://dx.doi.org/10.21037/mhealth-19-258). The series “Digital Health for Cardiovascular Disease” was commissioned by the editorial office without any funding or sponsorship. The authors have no other conflicts of interest to declare.
Ethical Statement: The authors are accountable for all aspects of the work in ensuring that questions related to the accuracy or integrity of any part of the work are appropriately investigated and resolved. Ethical permission to conduct the study has been approved by the ethics committee of the Garhwal Community Development and Welfare Society India and the Monash University Human Research Ethics Committee. This study will be conducted in accordance with the Declaration of Helsinki (as revised in 2013) and the Harmonized Tripartite Guideline for Good Clinical Practice from the International Conference on Harmonization; the Indian Council of Medical Research (ICMR); Guidelines for Biomedical Research in Human Subjects 2006, with any subsequent amendments; and all applicable laws of the Republic of India. All participating ASHAs and participants will sign an informed consent form which will be stored safely.
Open Access Statement: This is an Open Access article distributed in accordance with the Creative Commons Attribution-NonCommercial-NoDerivs 4.0 International License (CC BY-NC-ND 4.0), which permits the non-commercial replication and distribution of the article with the strict proviso that no changes or edits are made and the original work is properly cited (including links to both the formal publication through the relevant DOI and the license). See: https://creativecommons.org/licenses/by-nc-nd/4.0/.
References
- GBD 2016 Causes of Death Collaborators. Global, regional, and national age-sex specific mortality for 264 causes of death, 1980-2016: a systematic analysis for the Global Burden of Disease Study 2016. Lancet 2017;390:1151-210. [Crossref] [PubMed]
- World Health Organization. Noncommunicable diseases: Fact sheet (Updated June 2017). Accessed May 3 2020. Available online: http://www.who.int/mediacentre/factsheets/fs355/en/
- World Health Organization. Global Health Observatory (GHO) data - NCD mortality and morbidity. 2019. Accessed May 3 2020. Available online: https://www.who.int/gho/ncd/mortality_morbidity/en/
- Habib SH, Saha S. Burden of non-communicable disease: global overview. Diabetes Metab Syndr 2010;4:41-7. [Crossref]
- NCD Risk Factor Collaboration. Worldwide trends in blood pressure from 1975 to 2015: a pooled analysis of 1479 population-based measurement studies with 19.1 million participants. Lancet 2017;389:37-55. [Crossref] [PubMed]
- Gupta R, Gaur K, Ram C, et al. Emerging trends in hypertension epidemiology in India. J Hum Hypertens 2019;33:575-87. [Crossref] [PubMed]
- GBD 2013 Mortality and Causes of Death Collaborators. Global, regional, and national age-sex specific all-cause and cause-specific mortality for 240 causes of death, 1990-2013: a systematic analysis for the Global Burden of Disease Study 2013. Lancet 2014;385:17-171.
- Lim SS, Vos T, Flaxman AD, et al. A comparative risk assessment of burden of disease and injury attributable to 67 risk factors and risk factor clusters in 21 regions, 1990-2010: a systematic analysis for the Global Burden of Disease Study 2010. Lancet 2012;380:2224-60. [Crossref] [PubMed]
- Forouzanfar MH, Liu P, Roth GA, et al. Global burden of hypertension and systolic blood pressure of at least 110 to 115 mm Hg, 1990-2015. JAMA 2017;317:165-82. [Crossref] [PubMed]
- International Diabetes Federation. IDF Diabetes Atlas. Brussels, Belgium. 2011. Accessed May 3 2020. Available online: https://www.idf.org/e-library/epidemiology-research/diabetes-atlas/20-atlas-5th-edition.html
- Kim-Mitsuyama S, Soejima H, Yasuda O, et al. Anemia is an independent risk factor for cardiovascular and renal events in hypertensive outpatients with well-controlled blood pressure: a subgroup analysis of the ATTEMPT-CVD randomized trial. Hypertens Res 2019;42:883-91. [Crossref] [PubMed]
- Paul B, Wilfred NC, Woodman R, et al. Increased prevalence of anaemia in hypertension. Heart Lung Circ 2007;16:S82. [Crossref]
- Rweyemamu MM, Karimuribo ED, Mboera LE. The changing landscape for health research in Africa: the focus of the Southern African centre for infectious diseases and surveillance. Onderstepoort J Vet Res 2014;81:E1-2. [Crossref] [PubMed]
- Miranda JJ, Kinra S, Casas JP, et al. Non-communicable diseases in low-and middle-income countries: context, determinants and health policy. Trop Med Int Health 2008;13:1225-34. [Crossref] [PubMed]
- Bonita R, Magnusson R, Bovet P, et al. Country actions to meet UN commitments on non-communicable diseases: a stepwise approach. Lancet 2013;381:575-84. [Crossref] [PubMed]
- Willis-Shattuck M, Bidwell P, Thomas S, et al. Motivation and retention of health workers in developing countries: a systematic review. BMC health services research 2008;8:247. [Crossref] [PubMed]
- World Health Organization, PEPFAR & UNAIDS. Task shifting: rational redistribution of tasks among health workforce teams: global recommendations and guidelines. 2007. Accessed May 3 2020. Available online: https://apps.who.int/iris/handle/10665/43821
- Global Health Workforce Alliance, World Health Organization. Global experience of community health workers for delivery of health related millennium development goals: a systematic review, country case studies, and recommendations for integration into national health systems. 2010.
- Perry H, Zulliger R. How Effective Are Community Health Workers? An Overview of Current Evidence with Recommendations for Strengthening Community Health Worker Programs to Accelerate Progress in Achieving the Health-Related Millennium Development Goals. Johns Hopkins Bloomberg School of Public Health, 2012.
- Asia Pacific Observatory on Health Systems and Policies. Use of community health workers to manage and prevent noncommunicable diseases: policy options based on the findings of the COACH study. New Delhi: World Health Organization Regional Office for South-East Asia, 2019.
- Jeet G, Thakur J, Prinja S, et al. Community health workers for non-communicable diseases prevention and control in developing countries: evidence and implications. PLoS One 2017;12:e0180640. [Crossref] [PubMed]
- Rouse M, Charles M, DelVecchio A. Clinical decision support system (CDSS) TechTarget. 2020. Accessed May 3, 2020. Available online: https://searchhealthit.techtarget.com/definition/clinical-decision-support-system-CDSS
- Bennett JW, Glasziou PP. Computerised reminders and feedback in medication management: a systematic review of randomised controlled trials. Med J Aust 2003;178:217-22. [Crossref] [PubMed]
- Walton RT, Harvey E, Dovey S, et al. Computerised advice on drug dosage to improve prescribing practice. Cochrane Database Syst Rev 2001.CD002894. [Crossref] [PubMed]
- Kaushal R, Shojania KG, Bates DW. Effects of computerized physician order entry and clinical decision support systems on medication safety: a systematic review. Arch Intern Med 2003;163:1409-16. [Crossref] [PubMed]
- Bates DW, Teich JM, Lee J, et al. The impact of computerized physician order entry on medication error prevention. J Am Med Inform Assoc 1999;6:313-21. [Crossref] [PubMed]
- Shea S, DuMouchel W, Bahamonde L. A meta-analysis of 16 randomized controlled trials to evaluate computer-based clinical reminder systems for preventive care in the ambulatory setting. J Am Med Inform Assoc 1996;3:399-409. [Crossref] [PubMed]
- Balas EA, Weingarten S, Garb CT, et al. Improving preventive care by prompting physicians. Arch Intern Med 2000;160:301-8. [Crossref] [PubMed]
- Hunt DL, Haynes RB, Hanna SE, et al. Effects of computer-based clinical decision support systems on physician performance and patient outcomes: a systematic review. JAMA 1998;280:1339-46. [Crossref] [PubMed]
- Shiffman RN, Liaw Y, Brandt CA, et al. Computer-based guideline implementation systems: a systematic review of functionality and effectiveness. J Am Med Inform Assoc 1999;6:104-14. [Crossref] [PubMed]
- Mohan V, Deepa M, Pradeepa R, et al. Prevention of diabetes in rural India with a telemedicine intervention. J Diabetes Sci Technol 2012;6:1355-64. [Crossref] [PubMed]
- Mohan V, Sandeep S, Deepa R, et al. Epidemiology of type 2 diabetes: Indian scenario. Indian J Med Res 2007;125:217-30. [PubMed]
- Ministry of Health & Family Welfare. Hypertension: Screening, Diagnosis, Assessment, and Management of Primary Hypertension in Adults in India. Quick reference guide. Accessed 3 May 2020. Available online: http://clinicalestablishments.gov.in/WriteReadData/6591.pdf
- Brooke J. System Usability Scale (SUS): A quick and dirty usability scale. 1996.
- Abdel-All M, Thrift AG, Riddell M, et al. Evaluation of a training program of hypertension for accredited social health activists (ASHA) in rural India. BMC Health Serv Res 2018;18:320. [Crossref] [PubMed]
- National Health Mission, Ministry of Health & Family Welfare, Government of India. []. Operational Guidelines: Prevention, screening and control of common noncommunicable diseases. Accessed May 3 2020. Available online: https://nhm.gov.in
- Zaman S, Evans R, Singh R, et al. Questionnaire for the Arogya Sahyog pilot study. 2020. Accessed May 12 2020. Available online: https://figshare.com/articles/Questionnaire_for_the_Arogya_Sahyog_feasibility_study/12269579
- Browne RH. On the use of a pilot sample for sample size determination. Stat Med 1995;14:1933-40. [Crossref] [PubMed]
- Bangor A, Kortum PT, Miller JT. An empirical evaluation of the system usability scale. Int J Hum Comput Interact 2008;24:574-94. [Crossref]
- Kirkpatrick D, Kirkpatrick J. Evaluating training programs: The four levels. Berrett-Koehler Publishers, 2006.
- Geldsetzer P, Manne-Goehler J, Theilmann M, et al. Diabetes and Hypertension in India: A Nationally Representative Study of 1.3 Million Adults. JAMA Intern Med 2018;178:363-72. [Crossref] [PubMed]
- Surjaningrum ER, Jorm AF, Minas H, et al. Personal attributes and competencies required by community health workers for a role in integrated mental health care for perinatal depression: voices of primary health care stakeholders from Surabaya, Indonesia. Int J Ment Health Syst 2018;12:46. [Crossref] [PubMed]
Cite this article as: Zaman SB, Evans RG, Singh R, Singh A, Singh P, Singh R, Thrift AG. Feasibility of community health workers using a clinical decision support system to screen and monitor non-communicable diseases in resource-poor settings: study protocol. mHealth 2021;7:15.