mHealth for diabetes support: a systematic review of apps available on the Italian market
Introduction
Research on so called mHealth systems is rapidly developing, keeping pace with the speed at which technologies evolve and offer new instruments to perform all sorts of different actions (1,2). The area of chronic care is particularly interested in these new systems because of the characteristics of so called ‘non-communicable diseases’, which are closely correlated with risk factors such as smoking, alcohol abuse, unhealthy eating habits and sedentary lifestyle (3). Indeed, one of the hardest challenges for chronic care clinicians is the achievement of patient adherence to treatment and to healthy lifestyles. The problem with adherence is that it involves permanent behavior change (e.g., healthy lifestyles), something that is particularly difficult in the long term. It may be quite easy to perform a certain new behavior episodically; more difficult is to turn it into a permanent habit (4).
Due to this difficulty, the potentialities of technology as tools to support behavior change have been studied intensely in the past twenty years. With regard to chronic conditions in general, and diabetes in particular, the field of mHealth seems to offer promising solutions to facilitate various operations, such as: the collection of data regarding clinical parameters, the collection of information regarding habits and lifestyles, the sharing of information with clinicians or relevant others, making decisions, etc. (5). These possibilities cannot be underestimated. First, almost everybody nowadays owns a smartphone and carries it along everywhere; secondly, studies have shown the force of simple triggers on behavior change (6). In this context, the emerging field of positive technology is particularly relevant, i.e., “the scientific and applied approach to the use of technology for improving the quality of our personal experience through its structuring, augmentation, and/or replacement” (7). This new discipline is proposing insights into exactly which features of technological devices can impact positively on people’s behaviors and how these features can be integrated into instruments that are simultaneously usable, effective and tailored on the needs of specific target groups (7-9). Scholars have argued convincingly in favor of systems that are “context and situation aware”, i.e., able to take into account also the social and cognitive biases that individuals are subject to (10). These studies argue that technological devices are more likely to impact on behavior change when they are part of an integrated system that ‘nudges’ individuals towards certain behaviors, taking into consideration the larger context they are part of (11). Based on these studies, we assume that mHealth devices have a better chance of achieving the goal of supporting patient behavior change and self-management, when they are integrated in the ecology of the doctor-patient relationship and when they ‘suggest’ actions that are seamlessly woven into the pattern of patients’ everyday life (12).
In spite of being acknowledged as positive technology tools, the potential of mHealth devices as facilitators of permanent behavior change instead of mere channels for data or information exchange is yet controversial. Indeed, there is still lack of studies in which apps have been rigorously tested and it is not clear whether the ones currently on the market have been designed with the collaboration of clinicians, nor if they are based on reliable theoretical models regarding behavior change or decision making (5,13).
The main aim of this contribution is to present a review of the current offer of mHealth devices for diabetes support for the Italian-speaking public. The reason for focusing on this segment is that the review is a preparatory step towards the development and test of a mobile application to support diabetes patients in the context of a research project currently ongoing at Catholic University in Milan (Italy). The relevance of an app to support patient engagement and adherence to therapy and healthy behaviors is also explained by a recent report issued by the Italian Medicines Agency (AIFA), which shows that adherence to treatment and to healthy behaviors is far from optimal (14). Moreover, testing the app in the Italian context is considered as a first step towards an extension of the device also to other contexts. Finally, while there are some international reviews of research articles and reviews of mobile apps that discuss the role of technologies in diabetes self-management, to our knowledge there are no similar reviews for the Italian context. With respect to the review of research articles, the literature reveals a lack of data regarding Italy. When selecting articles on PubMed and Web of Science (keywords: diabetes AND Italy OR Italian AND app OR application; diabetes AND mobile AND Italy OR Italian), we did not obtain any positive results. We identified only five articles with Italian data, which were not studies on mobile apps (15,16), or studies that include interventions with type 2 diabetes patients (17-19).
Based on this review, we performed also a review of the apps on the Italian market. We are particularly interested to answer the following questions: how many apps are there?; what kind?; which functions do they have?; and especially, given the relevance of self-management and education oriented functionalities, how do these apps integrate these components?
Methods
Search criteria
For our review, we searched the apps available for the Italian-speaking public, downloaded and tested them. Apps were identified by one of the authors (MG Rossi) through a search in the Google Play Store (GPS) (for the Android mobile operating system) and Apple App Store (AAS) (for the IOS mobile operating system) during October 2015. We considered the first 20 entries on both stores for each of the following Italian keywords: “diabete” (diabetes), “diabete glicemia” (diabetes glycemia), “diabete educazione” (diabetes education), “diabete sport” (diabetes sport), “diabete movimento” (diabetes exercise), “diabete dieta” (diabetes diet), “diabete alimentazione” (diabetes nutrition).
We selected the apps using the following criteria.
Inclusion criteria:
- Apps that have Italian-language user interfaces;
- Apps used for blood glucose monitoring;
- Apps designed to be used primarily by patients (whether with or without the intervention of health care professionals, such as doctors, nurses, etc.);
- Apps designed (also) for patients with type 2 diabetes.
Exclusion criteria:
- Apps that have non-Italian user interfaces;
- Apps designed to be used by doctors and/or other care professionals;
- Apps that are not primarily designed for diabetes self-management (e.g., recipe apps; sport apps, such as pedometers);
- Apps that are not specifically designed for diabetes;
- Duplicated apps;
- Paid apps;
- Apps provided by healthcare facilities requiring login.
As discussed elsewhere, we have considered 140 apps on the GPS and 73 apps on the AAS (20). The smaller number of apps considered for IOS users probably depends on different inclusion criteria adopted by the two stores. Of the 180 rejected apps, 53.5% do not have an Italian-language user interface (67% for Android category and 25% for IOS category), 21.7% are paid apps (15% for Android category and 35% for IOS category) and 13.9% are not apps designed for the task of diabetes self-management (12.5% for Android category and 16.7% for IOS category). The total number of eligible apps is 20 for the GPS and 13 for the AAS; of which 16 are duplicate apps and have thus been left out. Overall, the final selection is of 17 apps (of which just 3 apps are available on both stores) [this description has appeared in the Letter to the Editor on the Journal of Diabetes Science and Technology, in which it was used to briefly discuss the limited educational functions of mHealth devices for people with diabetes in Italy (20)]. Figure 1 shows the details of this selection for both stores.
The test
Testing protocol
Both authors of this study installed the apps and uploaded real life glycemic profiles for one month. The profiles do not correspond to the parameters of a single individual, but have been prepared in collaboration with an expert clinician. Finally, the authors observed the apps’ responses by focusing on both verbal and visual dimensions.
Assessment criteria
We analyzed the reported usability and functions of the 17 selected apps. Specifically, we analyzed apps with respect to their educational level, since empirical evidence and clinical guidelines stress the relevance of education for the improvement of diabetes self-management, a mediator of adherence. In considering which of the apps’ functionalities to include in the category ‘educational’, we have decided to include those functions designated to offer information aimed at improving self-awareness and autonomy of patients regarding their disease. We have taken into account at least three different and complex dimensions: information giving, supporting critical thinking and developing practical skills (21-23). We have regarded education as a composite category and we have analyzed apps with reference to the following six sub-categories:
- Decision support: functions aimed at providing a feedback or a suggestion on medication dosage, food dosage, and so on.
- Messages: verbal messages used as alerts or reminders about therapy assumption or healthy behavior objectives (diet, exercise, water assumption, and so on).
- Contents: educational content about what is diabetes stricto sensu (its causes, consequences and functioning) and why it is important to have healthy lifestyles.
- Visual aids: functions aimed at providing a visual feedback on the trend of patients’ self-management. Examples from our review include: graphs, diagrams, statistics and tables.
- Goal setting: functions designed for planning activities and used by patients to work on a particular aspect of self-management.
- Social: functions designed for sharing ideas, doubts and information on social networks or social communities of diabetes patients.
Results
Table 1 shows the characteristics and functionalities of the tested apps. The first three columns describe technical information: for each app the table shows if it is available on the GPS, AAS or both of them; the version number; the average rating. The following eight columns are for data entry (blood glucose, exercise, diet, medication, blood pressure, weight, notes) and one for export or data sharing. Most of the apps allow the memorization of at least two categories from the data entry section. Only 23.5% of them (4 apps) allow to memorize (and share or export) all the different kinds of information (Figure 2).
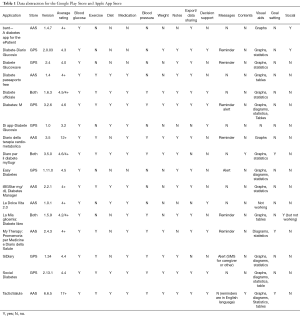
Full table
Results on the educational level are shown in Figure 3. Almost all apps (sixteen apps; 94.1%) feature one or more functions for the visual aids sub-category. Examples from our review include: graphs, diagrams, statistics and tables. Eight apps (47%) feature the decision support and message functions. Examples of decision support from our review include: different kinds of calculator or converter [from kg to check and vice versa, from mmol/L to mg/dL examples and vice versa, from mmol to mol-% and vice versa, body mass index (BMI) calculator, carbohydrate intake calculator, kilocalories calculator]; wellness registration: perception of individual wellness as expressed by images (emoticons) and words; symptom registration: annotation of perceived symptoms; communication with clinicians; tag: tagging of input data. However, only three apps (17.6%) feature the goal setting (for diet or exercise management) and social functions. In this latter case, examples from our review include the possibility to share ideas, doubts and information on Twitter, Facebook and Google Plus. Forums for discussion are not available for Italian speakers. And finally, just two apps (11.8%) feature the content function.
Discussion
International reviews have already underlined the lack of educational functions in the majority of mobile applications (24-26). In the same vein, also our review confirms this result for Italy. However, our review is different with respect to the method used for the analysis of the educational functions. As we mentioned above (section “Assessment criteria”), we considered six sub-categories for the conceptualization of what is an ‘educational component’. Following this conceptualization, the functionalities included in this category are the ones that support critical thinking by providing information as a trigger for action, as in the case of messages or decision support. When patients use, for example, a calculator to know the number of calories or carbohydrates, they can critically use this information to improve their self-management abilities. In the same vein, social communities might represent useful instruments to support forms of active learning; however, apps tested for this review do not allow this opportunity for Italian patients (if there are forums or web-sites linked to the apps, they are in English).
These considerations can be extended to other functions, related to both the verbal and the visual dimension of education. The case of visual aids is a very interesting example. As mentioned previously, 94.1% (16/17 apps) of the apps feature this function. However, from an educational point of view, the different visual choices (graphs, diagrams, statistics and tables) do not have the same value. Compare the three examples extracted from our reviewed apps (Figures 4,5,6). The diagram (Figure 4), the table with statistics (Figure 5) and the graphs (Figure 6) should represent, more or less, the same data (in most cases, data regard the values of blood glucose monitoring). However, the diagram (Figure 4) and the second graph (Figure 6B) seem to have a stronger education power: in these two cases, patients immediately see, interpret and know where their values are compared to where their values ought to be. On the contrary, other visual aids (e.g., statistics) do not seem as easy to understand and to interpret (27-30).
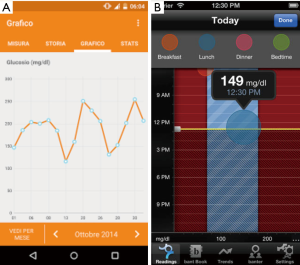
Finally, we also observed the linguistic structure of the messages used by the apps. We observed the messages aimed at providing information, along with messages aimed at improving self-awareness and autonomy of patients. While we believe that it is possible to use messages also in the latter sense, all messages evaluated are used to provide alerts or reminders. Our hypothesis is that this limited use of the verbal component might be due to a lack of theoretical models: in most cases, apps seem to be designed without reference to a clear theoretical and comprehensive model on how communication works. Scholars have already pointed out the lack of theoretical models regarding behavior change or decision making behind the development of most mHealth devices (10,31). Here we are stressing an even more specific lack of theoretical models regarding verbal communication for the construction of apps’ messages and contents.
Conclusions
The present review has some limitations, the most relevant being the fact that we were not able to test the paid apps, which are likely to feature more and more complex functionalities than the free ones.
However, even the fact that the free apps display such limited educational functionalities can be considered as a significant result. If it is true that diabetes is a disease affecting more seriously low-income levels of society worldwide, the tools to favor self-management and adherence should be available to the large public for free. For the specific case of the Italian market, the scarce offer of products might be partly explained by the still low digital literacy of the Italian public, in particular the over-65 users (32), which does not provide a sufficiently interesting market for developers or distributors of mHealth devices for patients. However, the consideration of the educational functionalities as mere instruments for the transmission of information suggests that app developers did not take into account more comprehensive conceptions of education, which include the ability to process and interpret information, allowing users to then act upon it.
More interdisciplinary studies seem necessary to reach this ambitious goal. In order to have apps with a stronger impact on users’ critical thinking skills as is requested for the aim of self-management, the design of mHealth devices should be based on solid theoretical models of education and communication. For this purpose, we are designing a new mobile app for diabetes patients by making extensive use of a cognitive-argumentative model of verbal communication (20,33). Based on this model’s theoretical assumptions, we are designing messages with different forms and functions, e.g., messages with an informative function, persuasive function, empowering function, and so on. Finally, our project is devoted to fill the gap in current research on mHealth for diabetes. In conjunction with the lack of solid results from international intervention studies on the efficacy of these devices on permanent behavior change, we have no results about intervention studies for Italy. For this purpose, we will verify the efficacy of the aforementioned model underlying our mHealth device by testing the app with type 2 diabetes patients in two hospitals in Italy. We will assess our mHealth intervention by verifying the improvement of patients’ awareness of their disease, self-management abilities (e.g., monitoring of HbA1c, correct performance of self-monitoring) and certainly app usability.
Further interventions are needed to collect data that will support the design of validated evidence-based educational functions for mHealth devices.
Acknowledgements
The study reported in this publication was supported by a grant from Catholic University of the Sacred Heart of Milano. The title of the grant is: “Progetto di ricerca d'interesse per l’Ateneo, Linea D.3.2, Anno 2014” for the project titled “Tecnologia Positiva e Healthy Ageing”, PI Giuseppe Riva. Preliminary results of this review have been presented at the 5th International conference on Applied Linguistics and Professional Practice (ALAPP), held at the University of Milano from 5–7 November 2015. We thank the audience at the conference for helpful comments on the paper.
Footnote
Conflicts of Interest: The authors have no conflicts of interest to declare.
References
- Oreskovic NM, Huang TT, Moon J. Integrating mHealth and Systems Science: A Combination Approach to Prevent and Treat Chronic Health Conditions. JMIR Mhealth Uhealth 2015;3:e62. [Crossref] [PubMed]
- Adibi S. editor. Mobile Health: A Technology Road Map (Vol. 5). New York: Springer, 2015.
- WHO. Global status report on noncommunicable diseases 2014. World Health 2014.176.
- Reach G. Can technology improve adherence to long-term therapies? J Diabetes Sci Technol 2009;3:492-9. [Crossref] [PubMed]
- Klonoff DC. The Current Status of mHealth for Diabetes: Will It Be the Next Big Thing? J Diabetes Sci Technol 2013;7:749-58. [Crossref] [PubMed]
- Fogg BJ. Persuasive Technology: Using Computers to Change What We Think and Do. Interactive Technologies 2002.
- Riva G, Baños RM, Botella C, et al. Positive Technology: Using Interactive Technologies to Promote Positive Functioning. Cyberpsychol Behav Soc Netw 2012;15:69-77. [Crossref] [PubMed]
- Riva G, Gaggioli A, Villani D, et al. Positive Technology for Healthy Living and Active Ageing. Stud Health Technol Inform 2014;203:44-56. [PubMed]
- Graffigna G, Barello S, Wiederhold BK, et al. Positive technology as a driver for health engagement. Stud Health Technol Inform 2013;191:9-17. [PubMed]
- Kaptein MC, Markopoulos P, de Ruyter B, et al. Persuasion in ambient intelligence. J Ambient Intell Humaniz Comput 2010;1:43-56. [Crossref]
- Hallsworth M, Berry D, Sanders M, et al. Stating appointment costs in SMS reminders reduces missed hospital appointments: Findings from two randomised controlled trials. PLoS One 2015;10:1-14.
- Eriksen S, Georgsson M, Hofflander M, et al. Health in hand: Putting mHealth design in context. 2014 IEEE 2nd International Workshop on Usability and Accessibility Focused Requirements Engineering, UsARE 2014–Proceedings. 2014:36-9.
- Georgsson M, Staggers N. Quantifying usability: an evaluation of a diabetes mHealth system on effectiveness, efficiency, and satisfaction metrics with associated user characteristics. J Am Med Inform Assoc 2016;23:5-11. [Crossref] [PubMed]
- Aifa. The Medicines Utilisation Monitoring Centre. National Report on Medicines use in Italy. Year 2014. Italian Medicines Agency 2015. Available online: Accessed 12.04.2017.www.agenziafarmaco.gov.it.
- Castelnuovo G, Manzoni GM, Cuzziol P, et al. TECNOB Study: Ad Interim Results of a Randomized Controlled Trial of a Multidisciplinary Telecare Intervention for Obese Patients with Type-2 Diabetes. Clin Pract Epidemiol Ment Health 2011;7:44-50. [Crossref] [PubMed]
- Lanzola G, Capozzi D, D’Annunzio G, et al. Going mobile with a multiaccess service for the management of diabetic patients. J Diabetes Sci Technol 2007;1:730-7. [Crossref] [PubMed]
- Rossi MC, Nicolucci A, Pellegrini F, et al. Interactive Diary for Diabetes: A Useful and Easy-to-Use New Telemedicine System to Support the Decision-Making Process in Type 1 Diabetes. Diabetes Technol Ther 2009;11:19-24. [Crossref] [PubMed]
- Rossi MC, Nicolucci A, Di Bartolo P, et al. Diabetes interactive diary: A new telemedicine system enabling flexible diet and insulin therapy while improving quality of life: An open-label, international, multicenter, randomized study. Diabetes Care 2010;33:109-15. [Crossref] [PubMed]
- Vuattolo O, Francescato MP, Della Mea V, et al. A smartphone application for preventing exercise-induced glycemic imbalances in type 1 diabetic patients. Stud Health Technol Inform 2012;180:1035-9. [PubMed]
- Rossi MG, Bigi S. Weak educational components in mHealth devices for diabetes support available on the Italian market. J Diabetes Sci Technol 2016;10:1199-200. [Crossref] [PubMed]
- Epstein RM, Street RL. The value of patient-centered care. Ann Fam Med 2011;9:100-3. [Crossref] [PubMed]
- Epstein RM, Gramling RE. What is shared in shared decision making? Complex decisions when the evidence is unclear. Med Care Res Rev 2013;70:94S-112S. [Crossref] [PubMed]
- Felton M, Crowell A, Liu T. Arguing to Agree: Mitigating My-Side Bias Through Consensus-Seeking Dialogue. Written Communication 2015;32:317-31. [Crossref]
- El-Gayar O, Timsina P, Nawar N, et al. Mobile applications for diabetes self-management: status and potential. J Diabetes Sci Technol 2013;7:247-62. [Crossref] [PubMed]
- Chomutare T, Fernandez-Luque L, Arsand E, et al. Features of mobile diabetes applications: review of the literature and analysis of current applications compared against evidence-based guidelines. J Med Internet Res 2011;13:e65. [Crossref] [PubMed]
- Brandell B, Ford C. Diabetes professionals must seize the opportunity in mobile health. J Diabetes Sci Technol 2013;7:1616-20. [Crossref] [PubMed]
- Kahneman D. Judgment and decision making: a personal view. Psychol Sci 1991;2:142-5. [Crossref]
- Gigerenzer G. Helping Doctors and Patients Make Sense of Health Statistics: Toward an Evidence-Based Society. Icots 2010;8:1-3.
- Gaissmaier W, Gigerenzer G. Statistical illiteracy undermines informed shared decision making. Z Evid Fortbild Qual Gesundhwes 2008;102:411-3. [Crossref] [PubMed]
- Reyna VF, Nelson WL, Han PK, et al. How numeracy influences risk comprehension and medical decision making. Psychol Bull 2009;135:943-73. [Crossref] [PubMed]
- Becker S, Miron-Shatz T, Schumacher N, et al. mHealth 2.0: Experiences, Possibilities, and Perspectives. JMIR Mhealth Uhealth 2014;2:e24. [Crossref] [PubMed]
- Istat Report “Noi-Italia”. 2015. Available online: Accessed 12.04.2017.http://noi-italia.istat.it.
- Bigi S. Key components of effective collaborative goal setting in the chronic care encounter. Commun Med 2014;11:103-15. [Crossref] [PubMed]
Cite this article as: Rossi MG, Bigi S. mHealth for diabetes support: a systematic review of apps available on the Italian market. mHealth 2017;3:16.