Influencing outcomes with automated time zero for sepsis through statistical validation and process improvement
Introduction
Each year in the United States more than 1.5 million people acquire sepsis and 250,000 die from sepsis (1). Sepsis is a multifaceted complication of infection that is life-threatening. Sepsis is typically conceptualized as a three-phase syndrome that progresses to severe sepsis which includes organ failure, diagnosed as septic shock or systemic inflammatory response syndrome (SIRS). The diagnosis of severe sepsis is difficult given its multiple diagnostic criteria, which generally includes altered mental status, abnormal vital signs, and organ dysfunction with a probable or confirmed infection (2). Clinicians providing inpatient care are especially vigilant in monitoring patients for sepsis given its prevalence; one in three people who die in a U.S. hospital have sepsis.
Given the severity of sepsis across the nation, the Surviving Sepsis Campaign (3) was started in 2002 with the goal of reducing mortality from sepsis by 25%. Seven strategies were designed to attain this objective including improving early diagnosis, increasing use of appropriate treatment guidelines, developing guidelines of care, and implementing a performance improvement program. The Surviving Sepsis Campaign was instrumental in the review and distribution of evidence-based guidelines (“sepsis bundles”) for the care of patients with sepsis, many of which are configured into inpatient electronic medical records (EMR) in the form of clinical decision support alerts and electronic order sets. Initiatives like the Surviving Sepsis Campaign provide tools for hospitals that publicly report sepsis process of care measures (4) on Medicare’s Hospital Compare portal (5) and set the standard for reportable events.
The reporting of timely implementation of evidence-based treatment guidelines, known as the SEP-1 bundle, is one such reportable process of care measure and involves the calculation of a starting point known as time zero. Time zero is defined as the time of presentation of the conditions of sepsis. Time zero may reflect the time of triage in the emergency department (ED) for someone who presents with sepsis or from documentation in the chart for someone who develops symptoms of sepsis while hospitalized. Typically, time zero is ascertained post-discharge by a team of abstractors who review the chart. Assigned retrospectively, time zero allows the abstractor to calculate the time when sepsis was first identified to when it was diagnosed and therapy was initiated. This project sought to improve upon the manual and post-hoc time zero by creating a real-time, automated time zero that could be used clinically during the hospital encounter to add value to patient care.
Like many health systems, ours has embarked on a multi-faceted program to improve the early identification of sepsis and initiate timely, evidence-based care to treat sepsis, two events that are associated with decreased sepsis mortality (6-10). This paper presents the post-hoc descriptive analysis of an innovative method designed and deployed to maximize positive predictive value, balancing the sensitivity and specificity of the EMR based sepsis alert using an automated time zero across 30 inpatient facilities within a single hospital system. The sepsis alert is visible in routine EMR workflow, displaying information about a patient’s condition in real-time through an application (app) developed specifically for this purpose using web technology by system developers. We aim to provide alerts to our clinicians that maximize positive predictive value while maintaining high sensitivity. When clinicians have confidence in the alerts that interrupt them, we believe their motivation to take action and therefore improve clinical outcomes is greatly enhanced. The addition of the automated time zero algorithm was hypothesized to improve measures of test performance, bundle compliance, intensive care unit length of stay, and mortality.
Methods
The current study represents a retrospective analysis of data collected during the normal course of patient care at a large, not-for-profit health system in the United States. It was deemed exempt from IRB review from an affiliated academic institution.
Sample & setting
These data reside in a data lake belonging to a faith-based system with 8,517 licensed acute care beds and 542 skilled nursing beds across 30 hospitals. The data platform was developed in cooperation with SAS (Cary, NC), the heart of which is a Cloudera (Palo Alto, CA, USA) instance of Hadoop® (Apache™ Software Foundation, Forest Hill, MD). The SAS platform allows for the ingestion of data from multiple source systems, including admission/discharge/transfer (ADT), ambulatory and inpatient electronic medical record, laboratory, patient satisfaction, population health, and health information exchange systems. Hadoop® provides open source, flat-file software that allows for the distributed processing of large datasets. A partnership with SAS provided the Visual Analytics™ tools to aid in data exploration and display. To date, the data lake contains one petabyte of information. The sample for this analysis was restricted to patients over the age of 16, cared for in 26 out of the 30 facilities, with a discharge date during the fiscal year 2017 (July 1, 2017 – June 30, 2018). Four facilities were excluded from this analysis because at the beginning of fiscal year 2017, two were not live on the EMR sepsis alert and two were not yet fully using the system-based Cerner EMR.
Study procedures
The St. John Sepsis Agent (11) was developed by Cerner Corporation (Kansas, MO) and served as the clinical decision support alert for sepsis in the EMR. It draws upon the best published evidence and uses cloud computing with big data analytics to screen high-risk patients and alert clinicians to the potential of sepsis. First, a statistical evaluation of the St. John Sepsis Agent was performed by installing and running the agent in silent mode in the production database. The goals of statistical analysis were to assess frequency and location of sepsis alerts as well as common measures of test performance (Table 1). Data were collected for a three-month period and correlated with actual discharge diagnoses of severe sepsis. A confusion matrix was created and a chart review was coordinated. All false negatives (no alert, sepsis diagnosis) and false positives (alert, no diagnosis) were manually reviewed and a sample of true positives (alert, sepsis diagnosis) and true negatives (no alert, no diagnosis) were also reviewed (Table 2). The PPV for the EMR sepsis alert was 38.0% (95% CI: 37.0–37.8%).

Full table
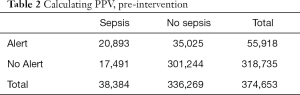
Full table
Next, a second algorithm was created to predict time zero. Time zero was defined as the presentation of severe sepsis using the following diagnostic codes: T81.12XA: post procedural septic shock, R65.20: severe sepsis without shock, or R65.21: severe sepsis with shock. It was calculated for every patient and may be the result of numerous presentations: (I) arrival in the emergency room with symptoms, (II) direct admission to the floor as a result of symptoms, (III) calculated from the time a clinician added a diagnosis to the problem list, or (IV) the last time stamp in the system where documentation of a suspected source of infection occurred alongside evidence of organ dysfunction (i.e., elevated lactate) and two SIRS criteria (12) (i.e., elevated heart rate, respiratory rate, abnormal temperature—fever or hypothermia, and an abnormal white blood cell count). It was based on the same criteria that coders use manually to abstract and report time zero to Centers for Medicare and Medicaid Services (CMS).
The current study measures the earliest period of time when the two algorithms were in use within the EMR (fiscal year 2017); the first generating a clinical decision support alert of possible sepsis and the second predicting time zero. The primary outcome of this analysis was the PPV after adding the second alert. Secondarily, alert timing, bundle compliance, intensive care unit (ICU) length of stay, and mortality from sepsis were measured.
Results
During the study period, a total of 55,918 sepsis alerts fired. Hospital location was ascertained for the majority of the alerts (n=55,780, 99.8%): 59.5% (33,174) fired in the emergency department, 19.9% (11,107) in the intensive care unit, and 20.6% (11,499) elsewhere in the hospital.
Measures of test performance
The PPV measured with the addition of the automated time zero was 52.1% (95% CI: 51.6–52.6%) (Table 3), representing a 39.4% increase when compared to the standard EMR based sepsis alert alone. Sensitivity and specificity analysis were performed with and without time zero (Figure 1). The sensitivity of the algorithm decreased slightly when time zero was added to the algorithm. The PPV of the alert increases (37.3% to 52.1%, P<0.001) when the time zero algorithm was added, increasing the likelihood of identifying cases of sepsis with the algorithm.
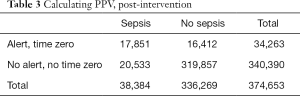
Full table
Alert timing
The cumulative line across the vertical axis (Figure 2) represents the number of sepsis cases during the study period, coded as severe sepsis or septic shock with an alert and a calculable time zero. Of the 11,889 alerts on patients subject to the SEP-1 measure with a calculable time zero, 9,172 (77%) occurred before the 180-minute deadline for sepsis bundle compliance, creating a window of opportunity for early diagnosis and treatment. Five thousand six hundred forty-one alerts (47%) fired within an hour of the calculated time zero, allowing for timely implementation of the sepsis resuscitation bundle.
Trends in bundle compliance, ICU length of stay, and mortality
SEP-1 bundle compliance was recorded at 50% (average) throughout the study period (Figure 3). This represents an increase of 14% (43.6–49.8%) from the previous year (z=9.84, P<0.0001). The average ICU length of stay for any patient with sepsis went from 45 to 41 hours, representing a 10% drop (t=4.91, P<0.0001). The mortality rate from all sepsis with both alerts during the study period decreased slightly, saving 48 lives.
Discussion
The addition of an automated time zero algorithm improved several key indicators including superior PPV, enhanced timing of the alert to allow for compliance with national standards for the early diagnosis and treatment of sepsis within a 180-minute window, improvements in bundle compliance, and reductions in ICU length of stay and mortality from sepsis.
An important element of our data science program is the ability to validate the tools selected for implementation. Instead of disseminating a vendor-supplied tool and trusting the math that informed its use elsewhere, we measured its performance in our own system, with our own cases before its distribution. This process is characterized by the Evidence Generating Medicine (EGM) model (13), powered by data science and biomedical informatics to systematically consider and incorporate research and quality improvement activities within an organization. EGM represents an evolution from traditional evidence-based medicine (EBM) models whereby the research to practice paradigm is unidirectional, such that clinicians go about the work of implementing the findings of researchers. An EGM model honors discoveries made at the point of care and, utilizing the volumes of data stored in electronic medical records and data lakes, aims to learn from practice level data, producing what Embi and colleagues call a “virtuous cycle of evidence generation, application, and refinement”.
The Institute of Medicine suggests that a learning healthcare system is better positioned to meet the quadruple aim objectives of improved population health and experience of care at lower costs, while attending to the well-being of healthcare providers. The engagement of data scientists and clinicians in sharing data, developing strategic goals, and developing quality improvement processes allowed our organization to learn how to better manage patients with sepsis. We suggest that the engagement of stakeholders with their own data in an EGM model resonates better with clinicians who can relate to learning from data they actively produced. We routinely share the PPV of our tools with clinicians and talk about the trustworthiness of alerts. We also continue to test our algorithms, investigating false positives and false negatives carefully to improve both alert performance and clinician confidence.
The automation of time zero improved the performance of the EMR-alone sepsis alert whereby the majority of sepsis cases were identified earlier. Early identification is critical to treatment and survivability as critical elements in the evidence-based sepsis care bundles can be implemented (these include things like starting intravenous fluid, administering antibiotics and tracking lactate levels). The Surviving Sepsis Campaign (SSC) first published guidelines for sepsis care in 2004. Revisions based on rigorous trials and EBM were introduced in 2008 and again in 2012. In 2015, CMS developed the SEP-1 measure, which monitored compliance with essential treatment based on the 2012 SSC bundle within a 180-minute window (14). In 2018, a 60-minute bundle was published by the SSC, leading many to anticipate a change in the CMS protocol requiring clinical response to a diagnosis of sepsis within a one-hour window. Almost half of our alerts with automated time zero occur before the 60-minute mark. Figure 4 presents the provider and administrator view of the app including the time since alert and time remaining notations, which are helpful in achieving these objectives. Improvements in the timing of the sepsis alert ensure that we are ready to adopt new standards and able to work towards continuous quality improvement related to the early diagnosis and treatment of sepsis. This focus on a reduced treatment window, whether regulated or not, places the focus of sepsis resuscitation efforts where it ought to be: the proper and timely delivery of evidence-based care to patients with sepsis in order to prevent mortality.
The associated reductions observed in ICU length of stay and mortality were expected with improvements in bundle compliance, in keeping with the literature (6-10). The cost savings derived from system financials were estimated at $1,500.00 per ICU day saved. The improvements in bundle compliance we detected were slow and steady over the observed study period, reflecting the intricoes and challenges in dissemination and implementation of best practices across a clinical enterprise. Anecdotally we can report that efforts to improve nurse-communication of sepsis alerts to physicians improved the timeliness of bundle compliance. A tremendous amount of effort is expended to educate clinicians about best practices and policies that facilitate early recognition of the signs and symptoms of sepsis. In the vast majority of cases, the implementation of SEP-1 bundles is dependent upon a physician order, which occurs only after a bedside nurse has notified the attending physician of the alert. The fields of translational informatics and implementation research have emerged to study the use of strategies to adopt and integrate evidence-based health interventions, moving from the “bench to the bedside” to improve the health of communities. This type of rigorous methodological study is needed to improve our ability to both distribute analytics tools and spread and sustain the evidence-based knowledge they support.
We were unable to locate another report on the innovation of an automated sepsis time zero in the literature. Others have reported variability in the inter-rater agreement of coders (15) reviewing charts post discharge for sepsis time zero, noting 36% agreement across three hospitals. A review (16) performed by physician chart reviewers post-discharge found a PPV of an EHR-embedded sepsis alert of 50.5%, but neither of these time zero assessments were automated nor available real-time during the inpatient encounter.
We acknowledge the following limitations in our study. This was a retrospective analysis and a report of pragmatic clinical practices, not a rigorous clinical trial. As such, we are unable to assess the multiple extraneous variables that may have influenced the timeliness of sepsis identification and bundle compliance. Multiple hospitals were included in the dataset, regardless of their go-live date, so long as they fell within the study window. This may reduce the impact of observed improvement as new facilities spend time learning the tools and order sets and developing processes to improve care with the new workflows. Like any post-hoc analysis, we are restricted to reporting the correlation of events. While internal validity may have been compromised, readers will appreciate the generalizability and be able to relate to the important clinical benefits we noted.
In conclusion, the automation of sepsis time zero improved the PPV of an existing EMR-based sepsis alert, which is important for improving the accuracy of the sepsis alert, thereby reducing false positive alert fatigue among clinical staff. The innovation of automated time zero also improved the timeliness of the EMR-based sepsis alert, which allowed for greater compliance with the life-saving SEP-1 resuscitation bundles within a 180-minute window. We believe this to be the first report of an automated time zero and suggest this improvement could be widely disseminated across other inpatient facilities. In the United States, mortality and morbidity from sepsis remains high and there is great variability in its diagnosis, management, and treatment (17), whereby patients presenting in medically underserved areas are less likely to receive proper treatment and more likely to succumb to death (18). Algorithms such as automated time zero may help to resolve these disparities in care in a variety of settings and reduce overall mortality.
Acknowledgments
The authors wish to acknowledge the advanced data science and IT teams at CommonSpirit Health: Srini Ganti, Brenda Downs, Sunil Kakade, Umesh Kalyanasundaram, Murali Nandula, Gaurav Padiyar, Harry Schned, Monica Spoerer.
Footnote
Conflicts of Interest: The authors have no conflicts of interest to declare.
Ethical Statement: The authors are accountable for all aspects of the work in ensuring that questions related to the accuracy or integrity of any part of the work are appropriately investigated and resolved. It was deemed exempt from IRB review from an affiliated academic institution.
References
- Hatfield KM, Dantes RB, Baggs J, et al. Assessing Variability in Hospital-Level Mortality Among U.S. Medicare Beneficiaries With Hospitalizations for Severe Sepsis and Septic Shock. Crit Care Med 2018;46:1753-60. [Crossref] [PubMed]
- Rhodes A, Evans LE, Alhazzani W, et al. Surviving Sepsis Campaign: International Guidelines for Management of Sepsis and Septic Shock 2016. Crit Care Med 2017;45:486-552. [Crossref] [PubMed]
- Society of Critical Care Medicine. Surviving Sepsis Campaign. 2018. Available online: http://www.survivingsepsis.org/About-SSC/Pages/History.aspx
- Centers for Medicare and Medicaid Services. Process of care measures reported under the Hospital Inpatient Quality Reporting (IQR) and Outpatient Quality Reporting (OQR) programs. 2019. Available online: medicare.gov/hospitalcompare/Data/Measures.html
- Centers for Medicare and Medicaid Services. Hospital Compare. 2019. Available online: www.medicare.gov/hospitalcompare/search.html
- Levy MM, Rhodes A, Phillips GS, et al. Surviving Sepsis Campaign: association between performance metrics and outcomes in a 7.5-year study. Crit Care Med 2015;43:3-12. [Crossref] [PubMed]
- Yokota PK, Marra AR, Martino MD, et al. Impact of appropriate antimicrobial therapy for patients with severe sepsis and septic shock--a quality improvement study. PLoS One 2014;9:e104475. [Crossref] [PubMed]
- Leisman DE, Goldman C, Doerfler ME, et al. Patterns and Outcomes Associated With Timeliness of Initial Crystalloid Resuscitation in a Prospective Sepsis and Septic Shock Cohort. Crit Care Med 2017;45:1596-606. [Crossref] [PubMed]
- Leisman D, Wie B, Doerfler M, et al. Association of Fluid Resuscitation Initiation Within 30 Minutes of Severe Sepsis and Septic Shock Recognition With Reduced Mortality and Length of Stay. Ann Emerg Med 2016;68:298-311. [Crossref] [PubMed]
- Scheer CS, Fuchs C, Kuhn SO, et al. Quality Improvement Initiative for Severe Sepsis and Septic Shock Reduces 90-Day Mortality: A 7.5-Year Observational Study. Crit Care Med 2017;45:241-52. [Crossref] [PubMed]
- Amland RC, Sutariya BB. Quick Sequential [Sepsis-Related] Organ Failure Assessment (qSOFA) and St. John Sepsis Surveillance Agent to Detect Patients at Risk of Sepsis: An Observational Cohort Study. Am J Med Qual 2018;33:50-7. [Crossref] [PubMed]
- Marik PE, Taeb AM. SIRS, qSOFA and new sepsis definition. J Thorac Dis 2017;9:943-5. [Crossref] [PubMed]
- Embi PJ, Payne PR. Evidence generating medicine: redefining the research-practice relationship to complete the evidence cycle. Med Care 2013;51:S87-91. [Crossref] [PubMed]
- Uppal A. The 1-hour sepsis bundle is serious - serious like a heart attack. Chest Physician. 2018. Available online: https://www.mdedge.com/chestphysician/article/184398/critical-care/1-hour-sepsis-bundle-serious-serious-heart-attack/page/0/1
- Rhee C, Brown SR, Jones TM, et al. Variability in determining sepsis time zero and bundle compliance rates for the centers for medicare and medicaid services SEP-1 measure. Infect Control Hosp Epidemiol 2018;39:994-6. [Crossref] [PubMed]
- Rolnick J, Downing NL, Shepard J, et al. Validation of test performance and clinical time zero for an electronic health record embedded severe sepsis alert. Appl Clin Inform 2016;7:560-72. [Crossref] [PubMed]
- Buchan CA, Bravi A, Seely AJ. Variability analysis and the diagnosis, management, and treatment of sepsis. Curr Infect Dis Rep 2012;14:512-21. [Crossref] [PubMed]
- Vakkalanka JP, Harland KK, Swanson MB, et al. Clinical and epidemiological variability in severe sepsis: an ecological study. J Epidemiol Community Health 2018;72:741-5. [Crossref] [PubMed]
Cite this article as: Colorafi KJ, Ferrell K, D’Andrea A, Colorafi J. Influencing outcomes with automated time zero for sepsis through statistical validation and process improvement. mHealth 2019;5:36.